Welcome to the Unsupervised Learning and Its Applications in Marketing course! In this course, you will delve into the fascinating world of unsupervised machine learning and its relevance to the field of marketing. Unsupervised learning is a powerful approach that allows us to uncover hidden patterns and insights from vast amounts of historical data without the need for explicit labels or human intervention. Through hands-on exercises and real-world examples, you will learn how to leverage the Python programming language to apply unsupervised learning algorithms in marketing contexts.

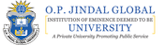
Unsupervised Learning and Its Applications in Marketing
This course is part of Machine Learning for Marketing Specialization
Taught in English

Instructor: Ambica Ghai
Included with
Course
Recommended experience
What you'll learn
Apply Python as an effective tool for implementing various algorithms.
Describe unsupervised learning and list its various algorithms.
List the various applications and promising areas for the application of unsupervised learning.
Skills you'll gain
Details to know

Add to your LinkedIn profile
36 quizzes
Course
Recommended experience
See how employees at top companies are mastering in-demand skills
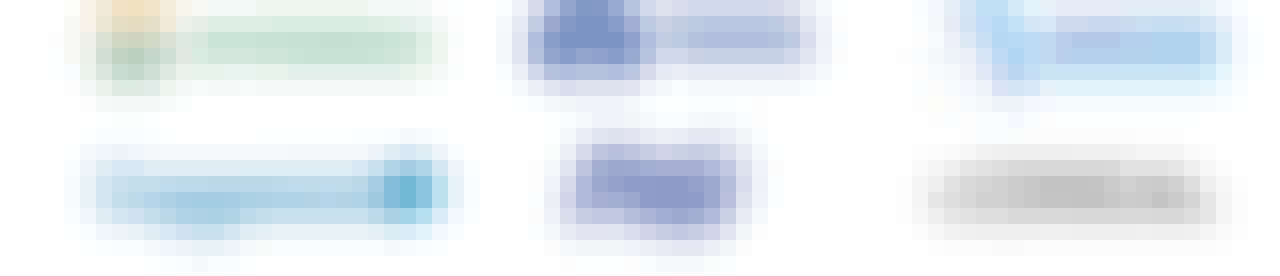
Build your subject-matter expertise
- Learn new concepts from industry experts
- Gain a foundational understanding of a subject or tool
- Develop job-relevant skills with hands-on projects
- Earn a shareable career certificate
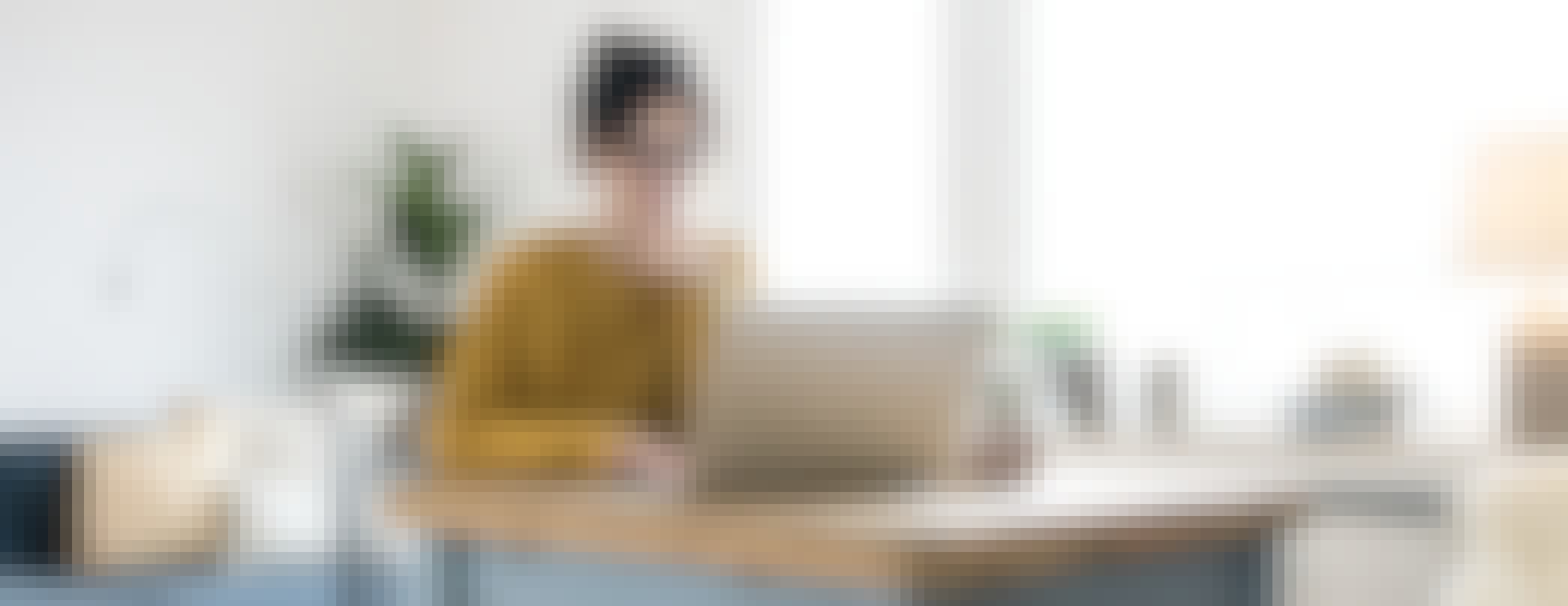
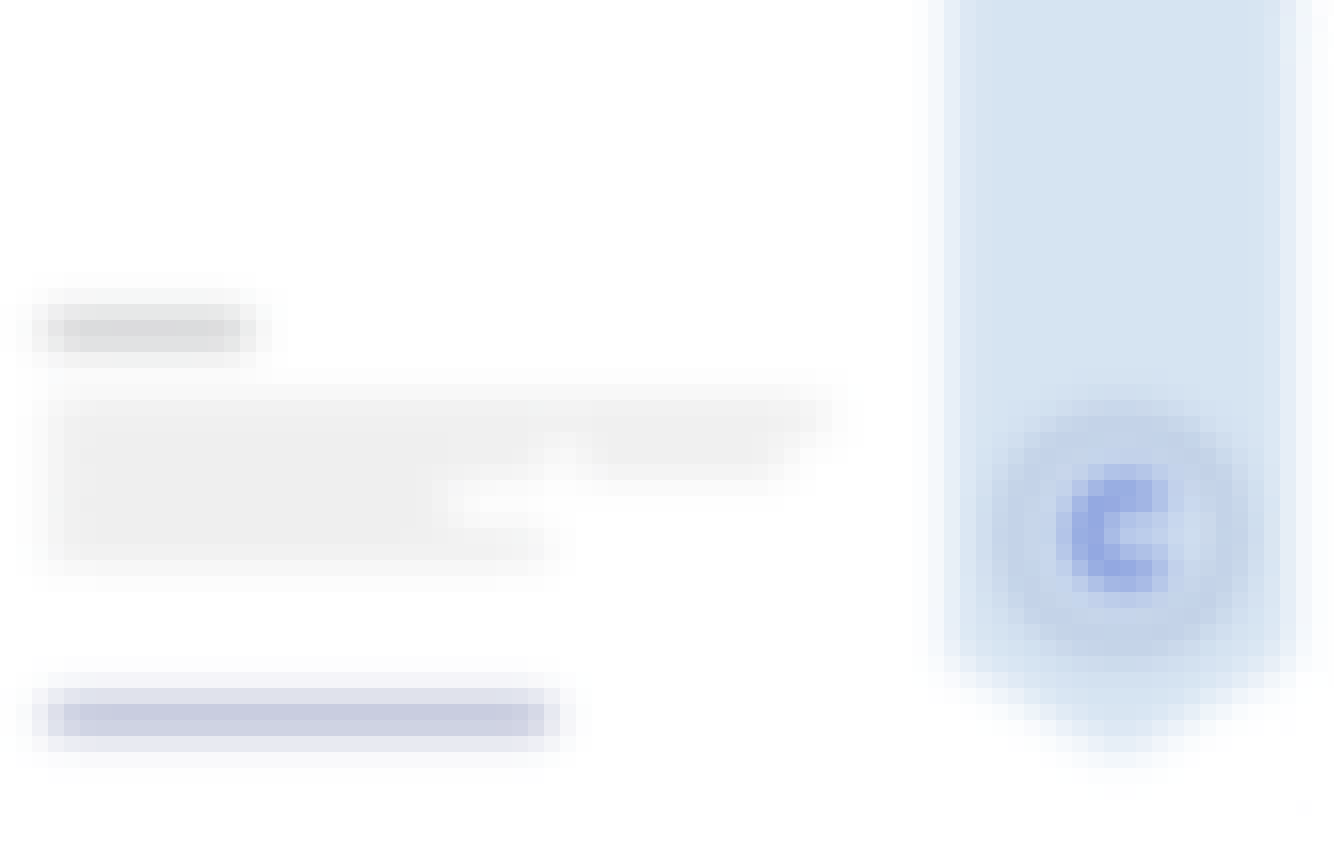
Earn a career certificate
Add this credential to your LinkedIn profile, resume, or CV
Share it on social media and in your performance review
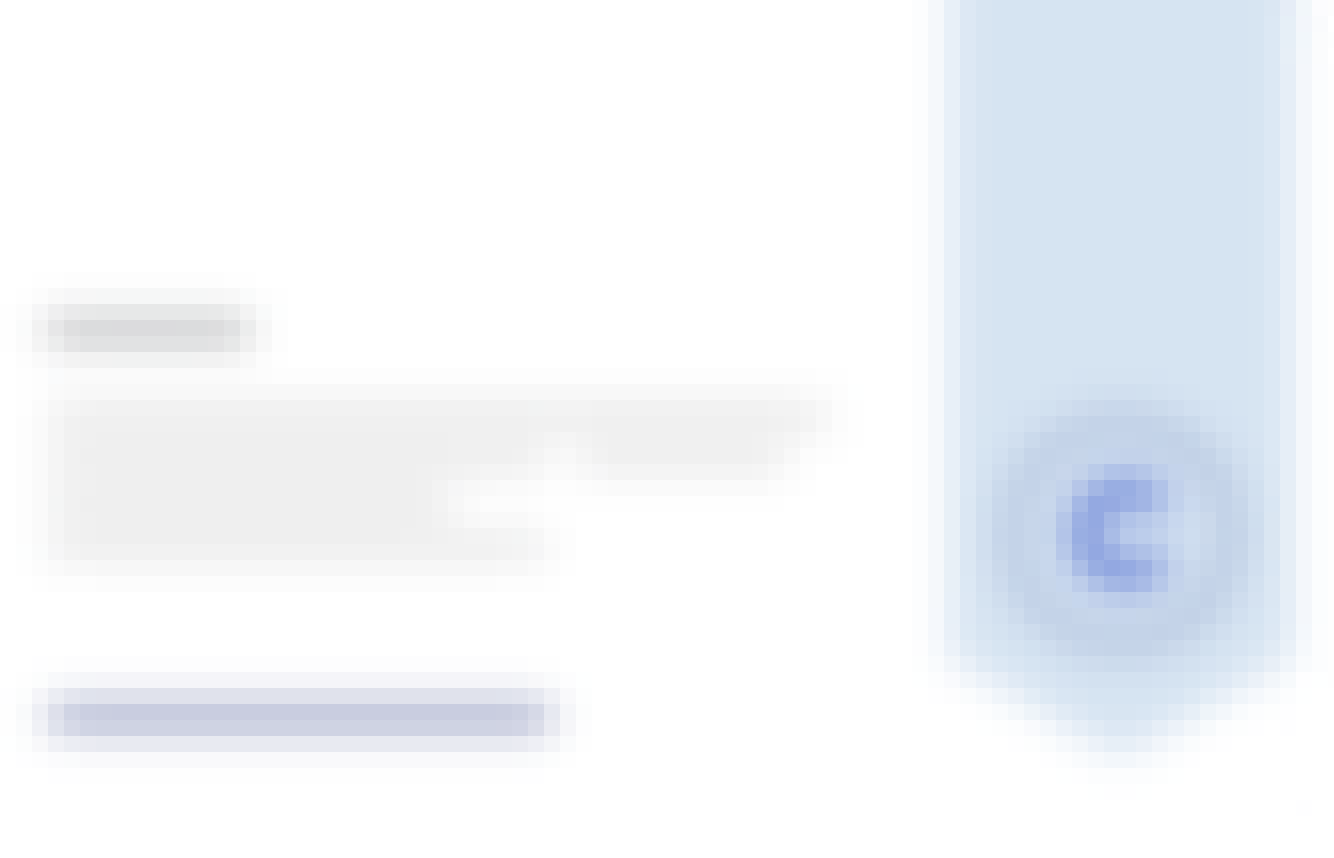
There are 12 modules in this course
In this module, you will be introduced to the exciting field of unsupervised learning and its applications in marketing. You will learn about various unsupervised learning algorithms and their functionalities, including clustering, dimensionality reduction, and association rule mining. Through hands-on exercises and practical examples, you will understand how these techniques can be used to uncover hidden patterns, identify customer segments, and gain valuable insights from large and complex marketing datasets. By the end of this module, you will have the knowledge and skills to apply unsupervised learning algorithms to solve marketing challenges, optimize campaigns, and make data-driven decisions that drive business growth. Get ready to unlock the potential of unsupervised learning and revolutionize your marketing strategies.
What's included
5 videos5 readings4 quizzes1 discussion prompt
This module provides a comprehensive introduction to clustering algorithms and their practical application using Python. You will gain a solid understanding of the fundamental concepts of clustering and explore different algorithms such as k-means, hierarchical clustering, and DBSCAN. Through hands-on exercises and coding examples, you will learn how to preprocess and transform data, select appropriate clustering algorithms based on data characteristics, and evaluate the performance of clustering models. Additionally, you will acquire the necessary skills to interpret and visualize clustering results, allowing you to gain valuable insights into patterns and structures within your data. By the end of this module, you will be equipped with the knowledge and practical experience to confidently apply clustering algorithms to real-world marketing datasets, enabling you to uncover meaningful clusters and make informed business decisions based on the extracted knowledge.
What's included
4 videos4 readings4 quizzes
This assessment is a graded quiz based on the modules covered this week.
What's included
1 quiz
In this module, you will dive into the fascinating world of customer segmentation and dimensionality reduction techniques. Customer segmentation allows you to divide your customer base into distinct groups based on shared characteristics, behaviors, or preferences. By understanding the unique needs and preferences of different customer segments, you can tailor your marketing strategies to effectively target and engage each segment. You will learn various clustering algorithms and techniques to perform customer segmentation using Python, enabling you to uncover meaningful insights about your customers and optimize your marketing efforts. Additionally, you will explore dimensionality reduction techniques, which are essential for dealing with high-dimensional data and extracting the most relevant features. Through hands-on exercises and real-world examples, you will gain practical skills in implementing customer segmentation and dimensionality reduction techniques to unlock valuable insights and drive marketing success.
What's included
4 videos4 readings4 quizzes1 discussion prompt
This module provides an opportunity to apply dimensionality reduction algorithms using Python. You will explore different types of dimensionality reduction algorithms, such as Principal Component Analysis (PCA), t-distributed stochastic neighbor embedding (t-SNE), and autoencoders. Through practical exercises and code implementations, you will gain hands-on experience in reducing the dimensionality of datasets, visualizing high-dimensional data in lower dimensions, and interpreting the results. Additionally, you will be introduced to anomaly detection techniques, which involve identifying rare or unusual data points that deviate from the norm. By the end of this module, you will have a solid understanding of dimensionality reduction algorithms and their application in real-world marketing scenarios, as well as the ability to detect anomalies effectively.
What's included
4 videos4 readings4 quizzes
This assessment is a graded quiz based on the modules covered this week.
What's included
1 quiz
In this module, you will delve into the practical aspects of anomaly detection by implementing various types of anomaly detection algorithms using Python. You will gain hands-on experience in applying algorithms such as statistical methods, clustering-based approaches, and machine learning-based techniques to detect anomalies in marketing data. Through step-by-step coding examples and guided exercises, you will learn how to preprocess data, select appropriate algorithms for different scenarios, tune parameters, and evaluate the performance of the models. By the end of this module, you will have a solid understanding of the implementation details of different anomaly detection algorithms and be equipped to apply them effectively in real-world marketing scenarios.
What's included
4 videos4 readings4 quizzes
Welcome to the module on Autoencoders and Association Learning! In this module, you will explore the fascinating field of autoencoders and its application in association learning, specifically in market basket analysis. In this module, you will learn how to apply autoencoders to extract meaningful features from data and use them to perform association learning using techniques such as the Apriori algorithm and FP-Growth algorithm. Through hands-on exercises and real-world examples, you will gain practical skills in implementing autoencoders and conducting association analysis to discover valuable insights from large-scale transactional data.
What's included
4 videos4 readings4 quizzes1 discussion prompt
This assessment is a graded quiz based on the modules covered this week.
What's included
1 quiz
In this module, you will delve into the world of semi-supervised learning. Semi-supervised learning is a powerful technique that combines the strengths of both supervised and unsupervised learning. It leverages a small amount of labeled data along with a large amount of unlabeled data to improve model performance. Through this module, you will gain an understanding of the concepts and principles behind semi-supervised learning. You will also learn how to implement semi-supervised learning algorithms using Python, enabling you to leverage the vast amounts of unlabeled data available in many real-world scenarios. By the end of this module, you will have the knowledge and skills to apply semi-supervised learning techniques in various domains, unlocking new opportunities for predictive modeling and data analysis.
What's included
4 videos4 readings4 quizzes1 discussion prompt
In this module, you will delve into the fascinating world of recommender systems and explore the concept of Boltzmann machines, which are powerful generative unsupervised models. You will gain a solid understanding of how Boltzmann machines work and their applications in recommendation systems. Through hands-on exercises and practical examples in Python, you will learn how to implement collaborative filtering using Boltzmann machines to make personalized recommendations. Additionally, this module will also touch upon the promising areas of unsupervised learning and provide insights into the future possibilities and advancements in the field. By the end of this module, you will be equipped with the knowledge and skills to build effective recommender systems and have a broader understanding of the potential of unsupervised learning in various domains.
What's included
4 videos4 readings4 quizzes
This assessment is a graded quiz based on the modules covered this week.
What's included
1 video1 quiz
Instructor

Offered by
Recommended if you're interested in Marketing
O.P. Jindal Global University
Google Cloud
O.P. Jindal Global University
O.P. Jindal Global University
Why people choose Coursera for their career




New to Marketing? Start here.

Open new doors with Coursera Plus
Unlimited access to 7,000+ world-class courses, hands-on projects, and job-ready certificate programs - all included in your subscription
Advance your career with an online degree
Earn a degree from world-class universities - 100% online
Join over 3,400 global companies that choose Coursera for Business
Upskill your employees to excel in the digital economy
Frequently asked questions
Access to lectures and assignments depends on your type of enrollment. If you take a course in audit mode, you will be able to see most course materials for free. To access graded assignments and to earn a Certificate, you will need to purchase the Certificate experience, during or after your audit. If you don't see the audit option:
The course may not offer an audit option. You can try a Free Trial instead, or apply for Financial Aid.
The course may offer 'Full Course, No Certificate' instead. This option lets you see all course materials, submit required assessments, and get a final grade. This also means that you will not be able to purchase a Certificate experience.
When you enroll in the course, you get access to all of the courses in the Specialization, and you earn a certificate when you complete the work. Your electronic Certificate will be added to your Accomplishments page - from there, you can print your Certificate or add it to your LinkedIn profile. If you only want to read and view the course content, you can audit the course for free.
If you subscribed, you get a 7-day free trial during which you can cancel at no penalty. After that, we don’t give refunds, but you can cancel your subscription at any time. See our full refund policy.