In this course, you will look at models and approaches that are designed to deal with challenges raised by time series data. The discussion covers the motivation for the use of particular models and the description of the characteristics of time series data, with a special attention raised to the potential memory. You will:

Give your career the gift of Coursera Plus with $160 off, billed annually. Save today.
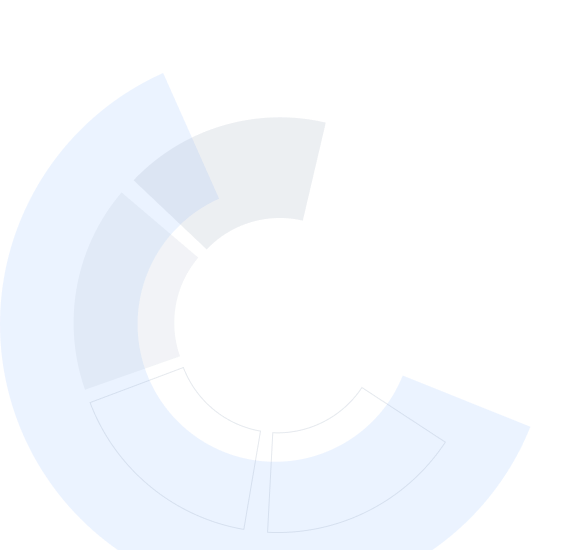
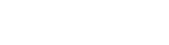
The Econometrics of Time Series Data
This course is part of Econometrics for Economists and Finance Practitioners Specialization

Instructor: Dr Leone Leonida
3,232 already enrolled
Included with
Recommended experience
What you'll learn
How to estimate the various model with R
How to check that the models are statistically valid with R
How to use the various models for decision making
Skills you'll gain
Details to know

Add to your LinkedIn profile
21 assignments
See how employees at top companies are mastering in-demand skills
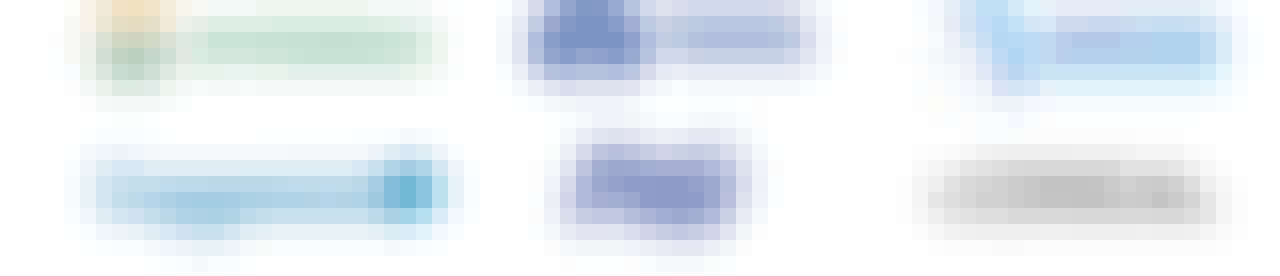
Build your subject-matter expertise
- Learn new concepts from industry experts
- Gain a foundational understanding of a subject or tool
- Develop job-relevant skills with hands-on projects
- Earn a shareable career certificate
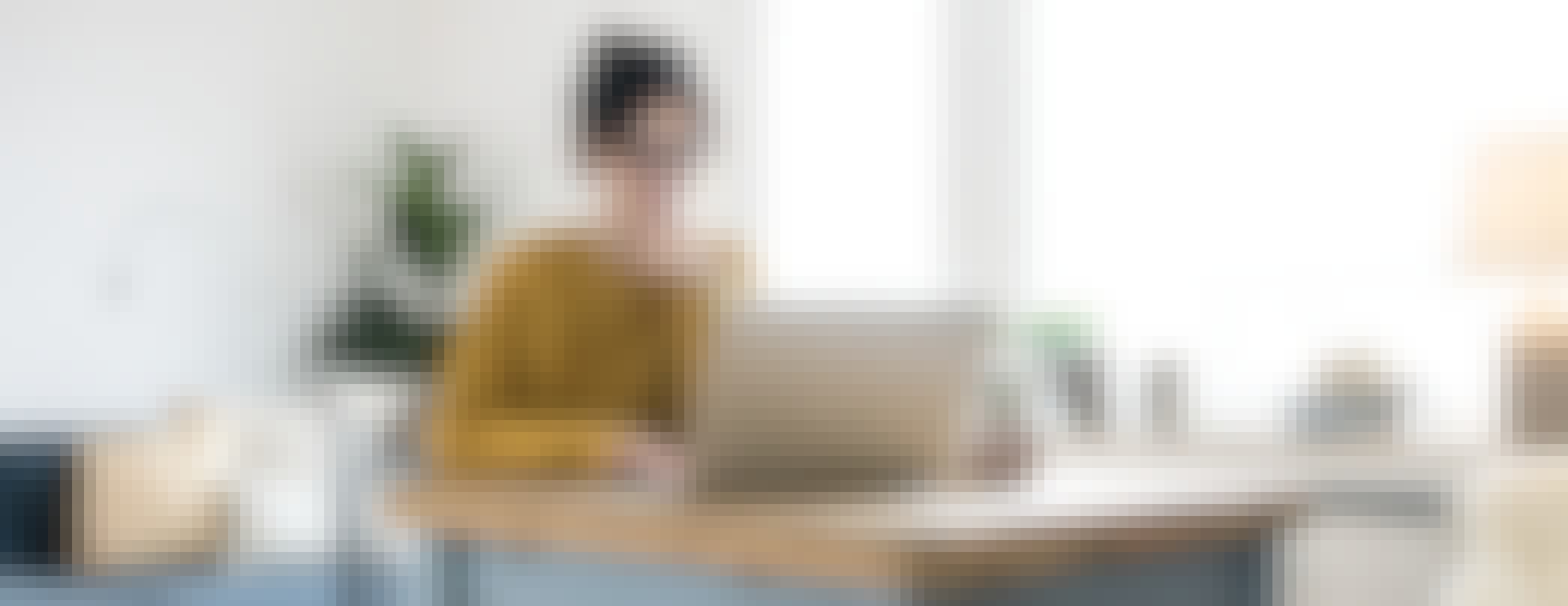
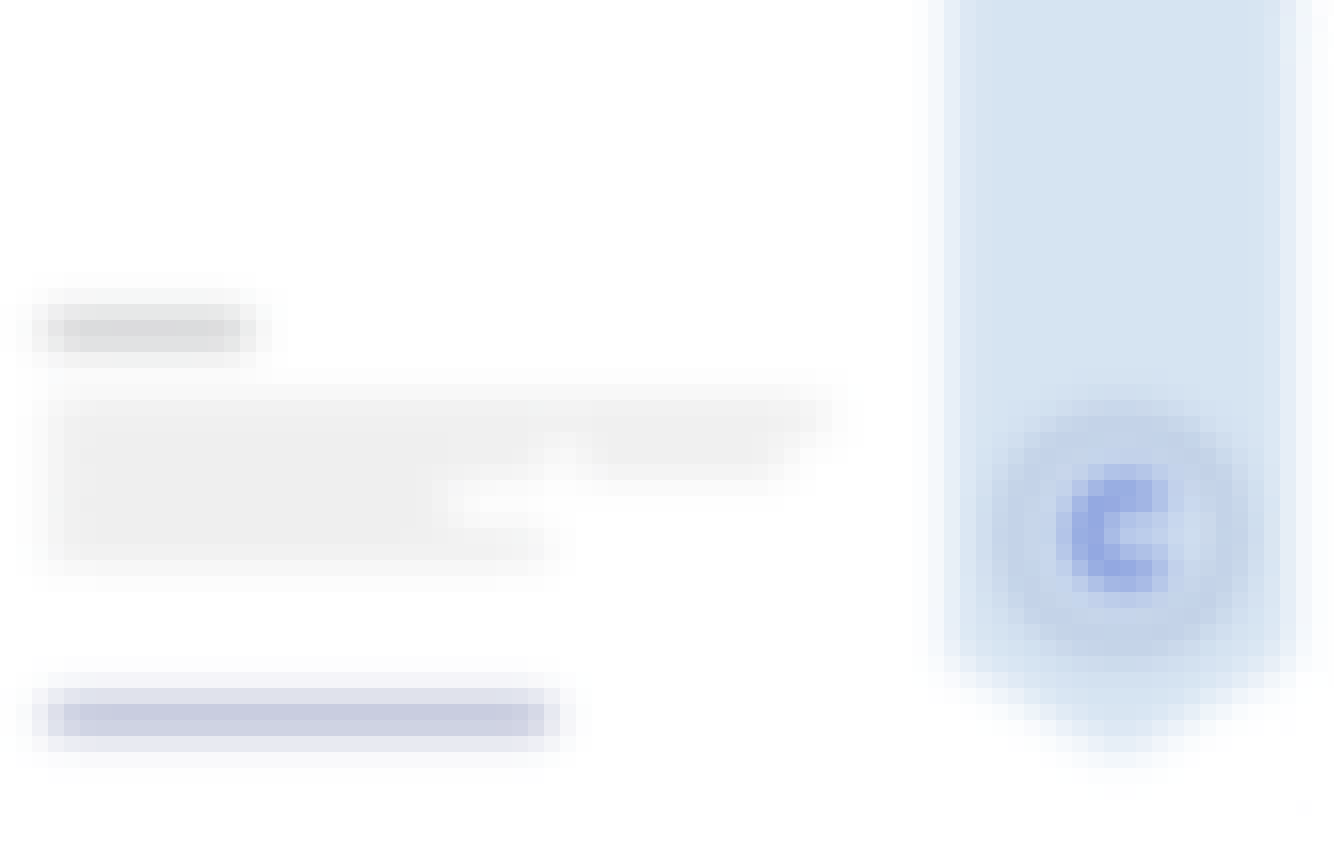
Earn a career certificate
Add this credential to your LinkedIn profile, resume, or CV
Share it on social media and in your performance review
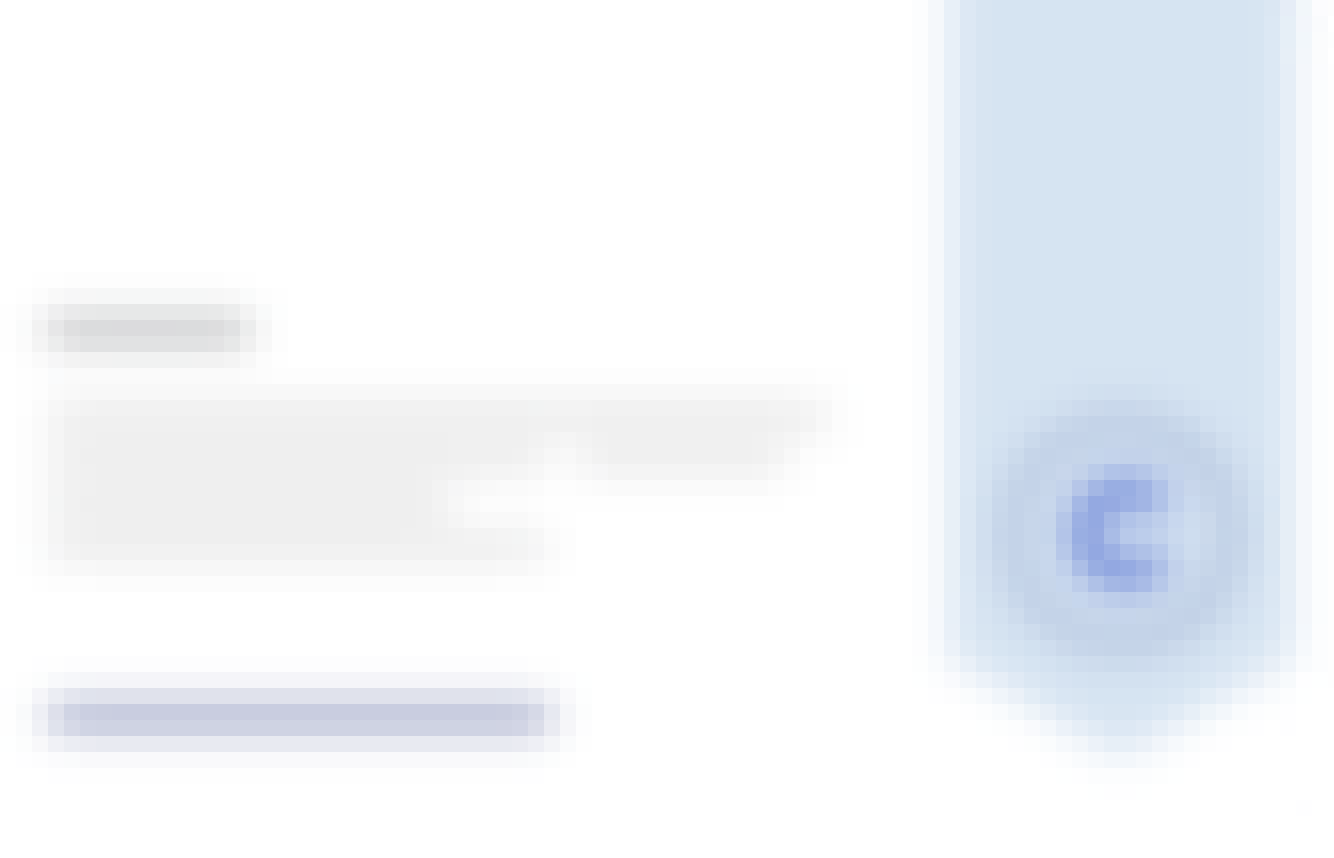
There are 4 modules in this course
This week’s materials present a number of time series observations. We look at white noise, trend stationary and non-stationary time series. We explore both at real observation about the GDP and to financial markets observations, and to generated series of data. We introduce both the idea of autocorrelation function and that of partial autocorrelation function as tools to understand the degree of persistency in a series of data.
What's included
5 videos6 readings5 assignments4 discussion prompts3 ungraded labs
This week we deal with stationary time series models. We present white noise, moving average, autoregression and autoregressive and moving average models. We describe the models and the different types of autocorrelation functions you have in each of these cases. We also discuss the problem of estimating the order of the autocorrelation and moving average models. We study the idea and the challenges raised by forecasting, and that’s raised by high persistency of the impact of shocks on the observed series.
What's included
4 videos6 readings6 assignments2 discussion prompts3 ungraded labs
This week we consider the problems raised by non-stationarity of time series observations. We define non-stationarity of time series data, and present the tests for non-stationarity, including the challenges raised by near non-stationarity, and that of potential correlation of the estimating model when testing for non-stationarity. We present a full example to show what are the consequences in cases where we adopt the classical linear regression model when observations are non-stationary. We introduce the idea of cointegration and present introductory models to test whether the variables are cointegrated.
What's included
4 videos4 readings5 assignments2 discussion prompts4 ungraded labs
This week’s materials discuss some stylised facts present across financial market returns, independent of the period, the financial tool and the market we study, that are volatility clustering and aggregational gaussianity. We discuss why these models, being nonlinear in nature, cannot be estimated via the classical linear regression model, and discuss and estimate some examples of autoregressive conditional heteroscedastic models. We discuss advantages and shortcomings of these models; building on the latter, we present some generalisation of the approach to generalised conditional heteroscedastic models (GARCH), GARCH-in-meena, TGARCH amd IGRACH models.
What's included
4 videos4 readings5 assignments1 peer review3 discussion prompts4 ungraded labs
Instructor

Offered by
Recommended if you're interested in Economics
Queen Mary University of London
Illinois Tech
The State University of New York
Erasmus University Rotterdam
Why people choose Coursera for their career





Open new doors with Coursera Plus
Unlimited access to 7,000+ world-class courses, hands-on projects, and job-ready certificate programs - all included in your subscription
Advance your career with an online degree
Earn a degree from world-class universities - 100% online
Join over 3,400 global companies that choose Coursera for Business
Upskill your employees to excel in the digital economy
Frequently asked questions
Access to lectures and assignments depends on your type of enrollment. If you take a course in audit mode, you will be able to see most course materials for free. To access graded assignments and to earn a Certificate, you will need to purchase the Certificate experience, during or after your audit. If you don't see the audit option:
The course may not offer an audit option. You can try a Free Trial instead, or apply for Financial Aid.
The course may offer 'Full Course, No Certificate' instead. This option lets you see all course materials, submit required assessments, and get a final grade. This also means that you will not be able to purchase a Certificate experience.
When you enroll in the course, you get access to all of the courses in the Specialization, and you earn a certificate when you complete the work. Your electronic Certificate will be added to your Accomplishments page - from there, you can print your Certificate or add it to your LinkedIn profile. If you only want to read and view the course content, you can audit the course for free.
If you subscribed, you get a 7-day free trial during which you can cancel at no penalty. After that, we don’t give refunds, but you can cancel your subscription at any time. See our full refund policy.