This advanced machine learning and deep learning course provides a robust foundation in these transformative technologies. Starting with an overview of deep learning, you'll explore its core concepts, real-world applications, and significance in AI's evolution.

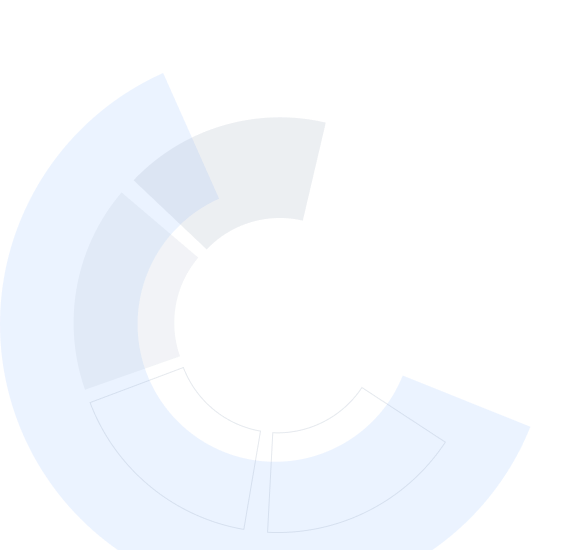
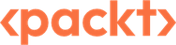
Advanced Machine Learning and Deep Learning
This course is part of R Ultimate 2023 - R for Data Science and Machine Learning Specialization

Instructor: Packt - Course Instructors
Included with
Recommended experience
What you'll learn
Identify and recall deep learning foundations and applications
Explain how to develop and train neural network models
Use techniques to evaluate and optimize model performance
Assess the effectiveness of CNNs for image processing and semantic segmentation
Details to know

Add to your LinkedIn profile
September 2024
4 assignments
See how employees at top companies are mastering in-demand skills
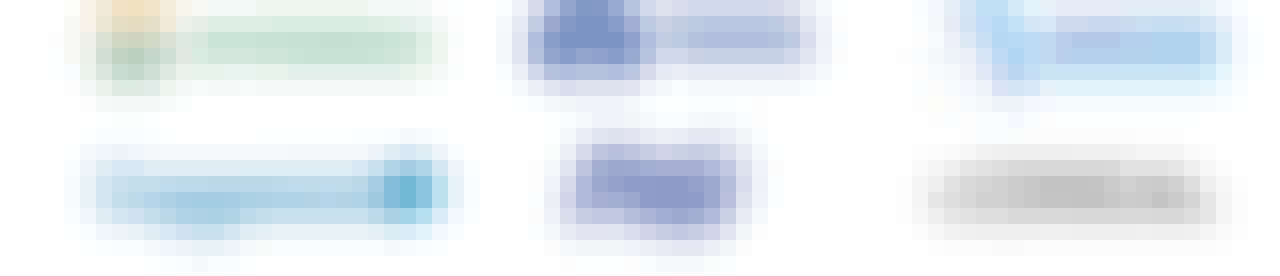
Build your subject-matter expertise
- Learn new concepts from industry experts
- Gain a foundational understanding of a subject or tool
- Develop job-relevant skills with hands-on projects
- Earn a shareable career certificate
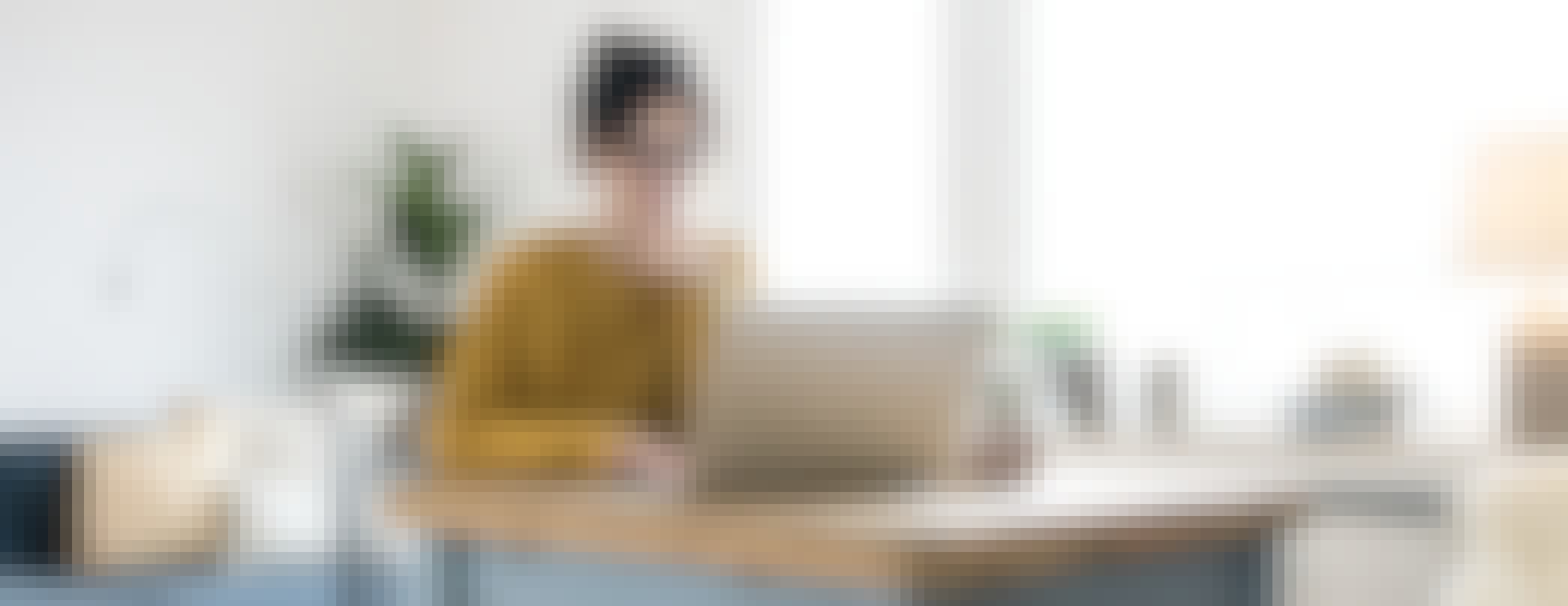
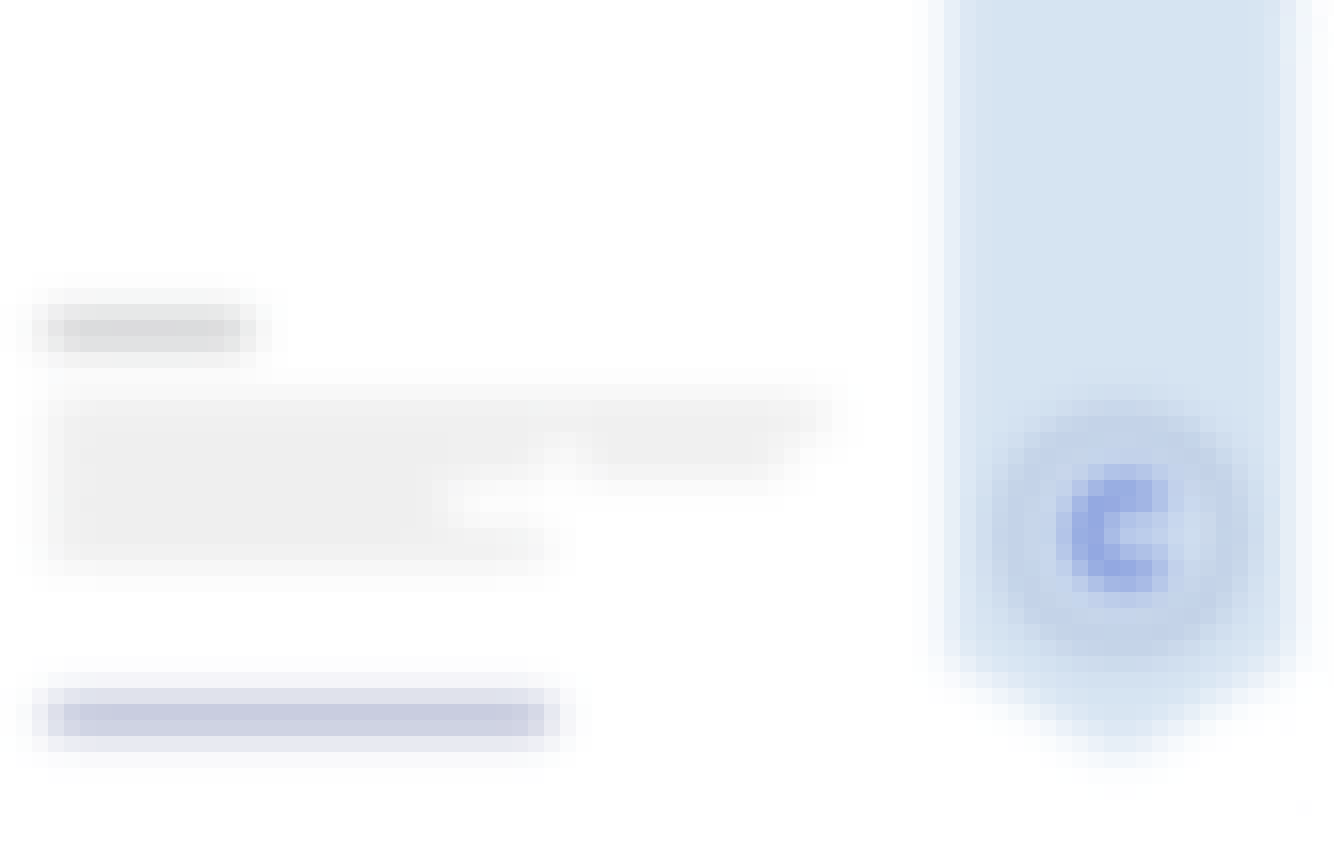
Earn a career certificate
Add this credential to your LinkedIn profile, resume, or CV
Share it on social media and in your performance review
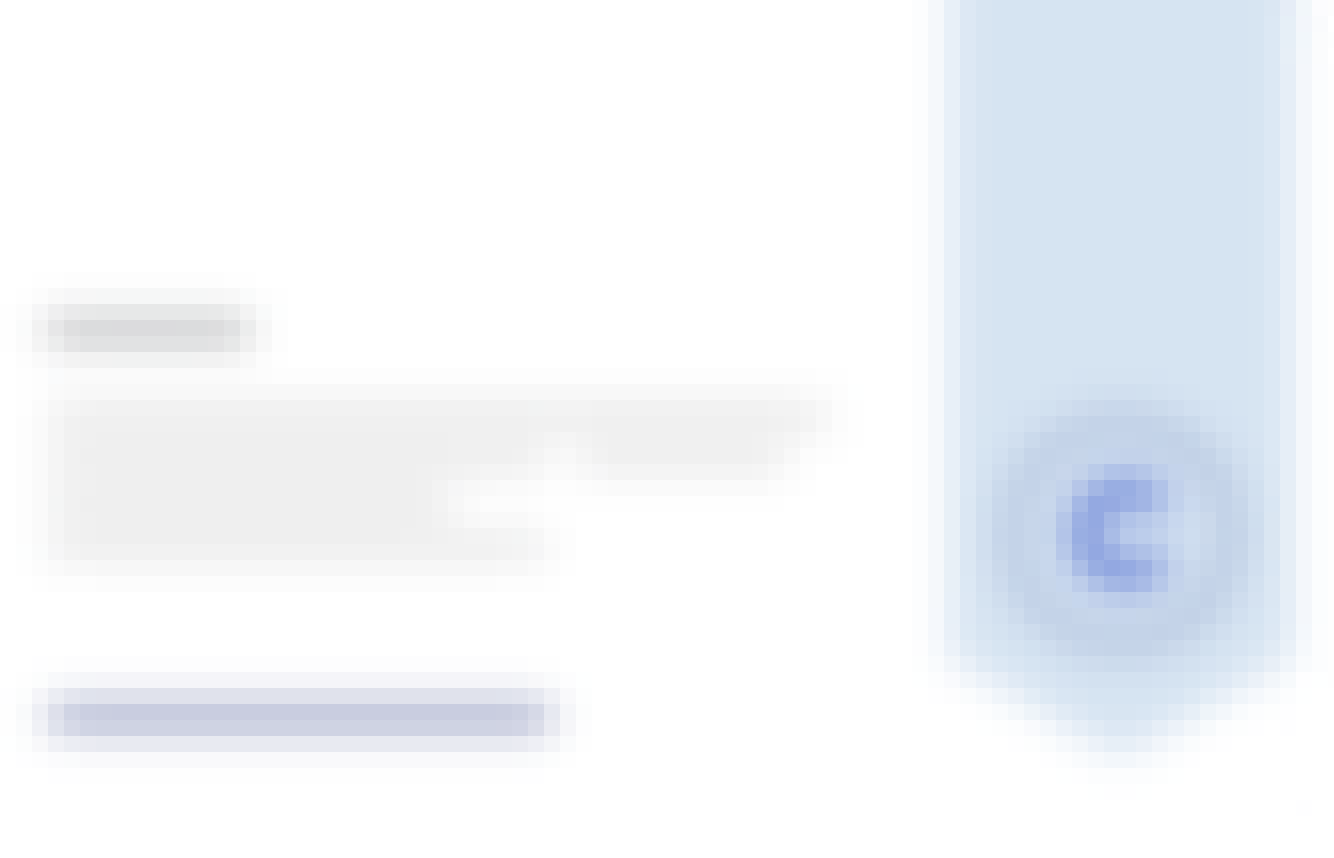
There are 8 modules in this course
In this module, we will explore the fundamental principles of deep learning, from its basic concepts to the intricacies of building and training neural networks. We will delve into various types of neural network layers, activation and loss functions, optimizers, and the tools and frameworks essential for deep learning development.
What's included
9 videos2 readings
In this module, we will delve into the specialized field of multi-target regression using deep learning. We will cover the theoretical foundations and follow a step-by-step coding guide to implement and refine regression models capable of predicting multiple continuous variables simultaneously.
What's included
3 videos
In this module, we will embark on a comprehensive journey into classification with deep learning, focusing on binary and multi-label classification techniques. We will build, code, and refine models that can effectively classify data into distinct or multiple categories, using hands-on labs and practical examples.
What's included
7 videos1 assignment
In this module, we will dive deep into Convolutional Neural Networks (CNNs), from their basic architecture to advanced applications. We will engage with interactive explorations, hands-on labs, and practical exercises to develop a robust understanding of CNNs' role in image recognition, classification, and semantic segmentation.
What's included
8 videos
In this module, we will explore the fascinating world of Autoencoders, focusing on their theoretical foundations and practical applications. We will learn how to effectively implement Autoencoders, understand their diverse uses, and gain hands-on experience through coding labs.
What's included
3 videos
In this module, we will delve into transfer learning and pretrained models, exploring how these techniques revolutionize the efficiency and effectiveness of deep learning. We will learn to apply these methods practically through lab sessions, significantly enhancing our deep learning projects.
What's included
3 videos1 assignment
In this module, we will explore Recurrent Neural Networks (RNNs) and their application in processing sequential data. We will focus on Long Short-Term Memory (LSTM) networks for time series prediction, gaining practical experience through coding labs and hands-on experimentation.
What's included
5 videos
In this module, we will explore Shiny, a framework for building interactive web applications. We will learn about its essential components, delve into language selection and reactive expressions, and gain hands-on experience in developing and deploying Shiny apps for real-world use.
What's included
10 videos1 reading2 assignments
Instructor

Offered by
Recommended if you're interested in Data Analysis
DeepLearning.AI
University of Pennsylvania
Why people choose Coursera for their career




New to Data Analysis? Start here.

Open new doors with Coursera Plus
Unlimited access to 10,000+ world-class courses, hands-on projects, and job-ready certificate programs - all included in your subscription
Advance your career with an online degree
Earn a degree from world-class universities - 100% online
Join over 3,400 global companies that choose Coursera for Business
Upskill your employees to excel in the digital economy
Frequently asked questions
Yes, you can preview the first video and view the syllabus before you enroll. You must purchase the course to access content not included in the preview.
If you decide to enroll in the course before the session start date, you will have access to all of the lecture videos and readings for the course. You’ll be able to submit assignments once the session starts.
Once you enroll and your session begins, you will have access to all videos and other resources, including reading items and the course discussion forum. You’ll be able to view and submit practice assessments, and complete required graded assignments to earn a grade and a Course Certificate.