In this course you’ll explore how to turn promising ML prototypes into robust, scalable, and maintainable systems that deliver real value. Through hands-on demos, practical tools, and real-world case studies from companies like Netflix, Uber, and Google, you’ll gain a comprehensive understanding of what it takes to run ML systems effectively in production using MLOps.

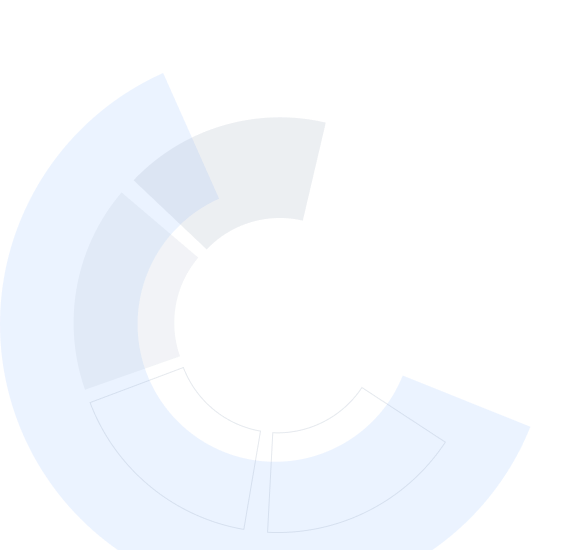
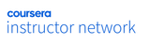
Recommended experience
What you'll learn
Implement scalable MLOps workflows that ensure efficient and reliable machine learning operations.
Build CI/CD pipelines for seamless and automated model updates, streamlining the development lifecycle.
Monitor deployed ML models for performance and drift.
Optimize AI infrastructure to handle scalability challenges and support high-performance deployments.
Skills you'll gain
- IT Infrastructure
- Containerization
- MLOps (Machine Learning Operations)
- Infrastructure Architecture
- Artificial Intelligence and Machine Learning (AI/ML)
- Real Time Data
- Scalability
- CI/CD
- Continuous Integration
- Data Infrastructure
- Continuous Deployment
- DevOps
- Continuous Monitoring
- Version Control
- Kubernetes
- Docker (Software)
- Cloud Infrastructure
Details to know

Add to your LinkedIn profile
March 2025
1 assignment
See how employees at top companies are mastering in-demand skills
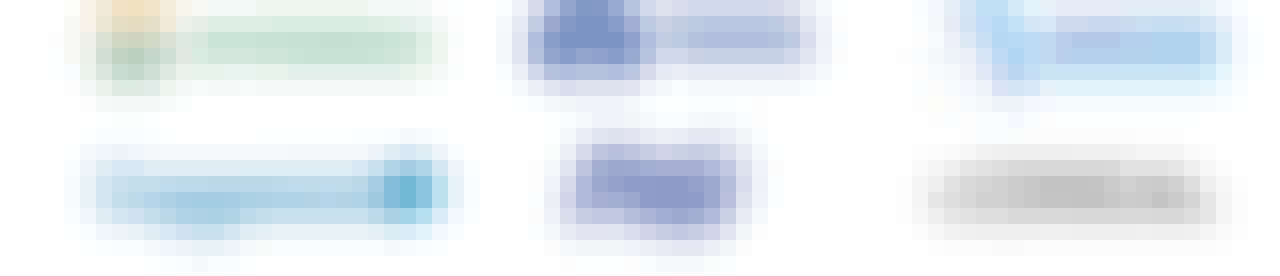
There is 1 module in this course
In this course, you’ll explore how to turn promising ML prototypes into robust, scalable, and maintainable systems that deliver real value. Through hands-on demos, practical tools, and real-world case studies from companies like Netflix, Uber, and Google, you’ll gain a comprehensive understanding of what it takes to run ML systems effectively in production using MLOps.
What's included
11 videos7 readings1 assignment1 peer review2 discussion prompts
Earn a career certificate
Add this credential to your LinkedIn profile, resume, or CV. Share it on social media and in your performance review.
Offered by
Explore more from Machine Learning
- Status: Free Trial
Duke University
- Status: Free Trial
- Status: Preview
- Status: Free Trial
Duke University
Why people choose Coursera for their career





Open new doors with Coursera Plus
Unlimited access to 10,000+ world-class courses, hands-on projects, and job-ready certificate programs - all included in your subscription
Advance your career with an online degree
Earn a degree from world-class universities - 100% online
Join over 3,400 global companies that choose Coursera for Business
Upskill your employees to excel in the digital economy
Frequently asked questions
Access to lectures and assignments depends on your type of enrollment. If you take a course in audit mode, you will be able to see most course materials for free. To access graded assignments and to earn a Certificate, you will need to purchase the Certificate experience, during or after your audit. If you don't see the audit option:
The course may not offer an audit option. You can try a Free Trial instead, or apply for Financial Aid.
The course may offer 'Full Course, No Certificate' instead. This option lets you see all course materials, submit required assessments, and get a final grade. This also means that you will not be able to purchase a Certificate experience.
When you purchase a Certificate you get access to all course materials, including graded assignments. Upon completing the course, your electronic Certificate will be added to your Accomplishments page - from there, you can print your Certificate or add it to your LinkedIn profile. If you only want to read and view the course content, you can audit the course for free.
You will be eligible for a full refund until two weeks after your payment date, or (for courses that have just launched) until two weeks after the first session of the course begins, whichever is later. You cannot receive a refund once you’ve earned a Course Certificate, even if you complete the course within the two-week refund period. See our full refund policy.
More questions
Financial aid available,