This course covers practical algorithms and the theory for machine learning from a variety of perspectives. Topics include supervised learning (generative, discriminative learning, parametric, non-parametric learning, deep neural networks, support vector Machines), unsupervised learning (clustering, dimensionality reduction, kernel methods). The course will also discuss recent applications of machine learning, such as computer vision, data mining, natural language processing, speech recognition and robotics. Students will learn the implementation of selected machine learning algorithms via python and PyTorch.

Heat up your career with 40% off top courses from Google, Adobe, and more. Save today.
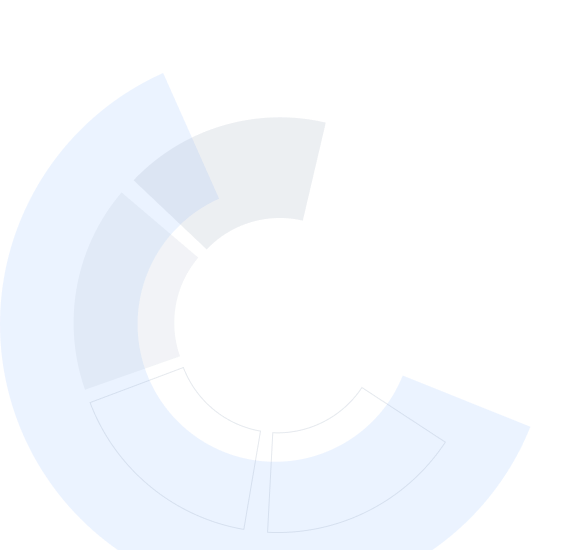
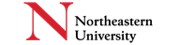
Machine Learning for Engineers: Algorithms and Applications

Instructor: Qurat-ul-Ain Azim
Included with
Skills you'll gain
- Statistical Analysis
- Deep Learning
- Dimensionality Reduction
- Machine Learning
- Unsupervised Learning
- Statistical Methods
- Applied Machine Learning
- Complex Problem Solving
- Supervised Learning
- Machine Learning Algorithms
- Algorithms
- PyTorch (Machine Learning Library)
- Statistical Modeling
- Regression Analysis
- Statistical Machine Learning
- Predictive Modeling
- Artificial Intelligence and Machine Learning (AI/ML)
Details to know

Add to your LinkedIn profile
January 2025
7 assignments
See how employees at top companies are mastering in-demand skills
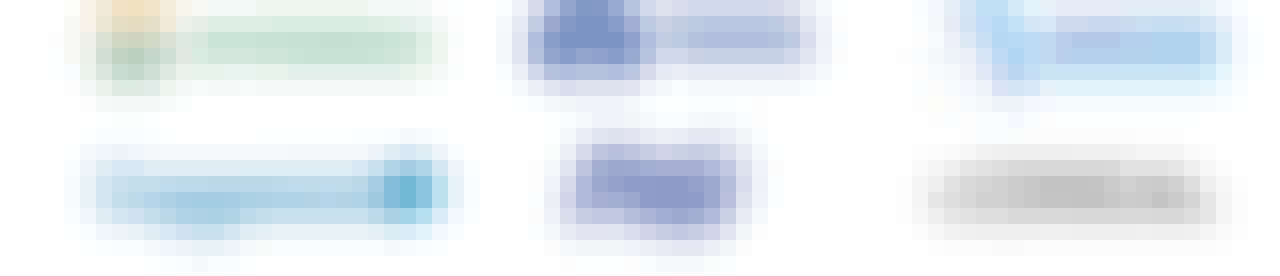
There are 4 modules in this course
This week provides an introduction to the field of statistical learning, exploring its scope and practical applications across various domains. Students will analyze how statistical learning techniques are used to make predictions, infer relationships, and uncover patterns in complex datasets. The module also offers a review of the key concepts essential for success in the course, including statistical models, data handling, and learning algorithms. By the end of the module, you will have a solid understanding of statistical learning principles and be prepared to apply them in real-world scenarios, laying the foundation for deeper exploration in machine learning and data science.
What's included
1 video7 readings1 assignment1 discussion prompt
This week introduces you to the concept of Maximum Likelihood Estimation (MLE) and its application in statistical modeling. You will gain a thorough understanding of how to mathematically implement MLE and apply it to real-world datasets. The week will revisit foundational concepts of convex optimization, offering a solid foundation in optimization techniques. Additionally, the iterative process of the gradient descent algorithm will be explored, allowing you to understand and implement this method for finding optimal solutions in machine learning models. Through a combination of theoretical knowledge and practical application, you will build essential skills in statistical estimation and optimization, preparing for advanced studies in machine learning and data analysis.
What's included
2 videos3 readings2 assignments2 discussion prompts
In this module, you will gain a comprehensive understanding of supervised machine learning from model training to evaluation. You’ll interpret each step in the learning process and apply training and evaluation techniques to real-world data. This will enable you to fit and assess models, while addressing issues like overfitting and underfitting. By exploring the bias-variance trade-off, you can optimize models for greater accuracy and reliability. Cross-validation methods are also covered, equipping students with robust tools for model assessment and performance analysis. This week will combine theoretical insights preparing you for the advanced work in machine learning.
What's included
2 videos4 readings2 assignments
This module, we will focus on the foundational principles of linear regression, a key technique in predictive modeling. You will learn to apply linear regression models and derive the ordinary least squares (OLS) formulation, gaining insight into how OLS is used to fit data accurately. We will also cover solution methods, including gradient descent and convex optimization, which provides a toolkit for efficient model training. You will explore regularization techniques to enhance model robustness and prevent overfitting. By implementing these regularized regression models in Python, you will gain hands-on experience in model optimization.
What's included
2 videos2 readings2 assignments1 discussion prompt
Earn a career certificate
Add this credential to your LinkedIn profile, resume, or CV. Share it on social media and in your performance review.
Instructor

Offered by
Explore more from Algorithms
- Status: Free Trial
Politecnico di Milano
- Status: Free Trial
Johns Hopkins University
The University of Chicago
- Status: Free Trial
University of Washington
Why people choose Coursera for their career





Open new doors with Coursera Plus
Unlimited access to 10,000+ world-class courses, hands-on projects, and job-ready certificate programs - all included in your subscription
Advance your career with an online degree
Earn a degree from world-class universities - 100% online
Join over 3,400 global companies that choose Coursera for Business
Upskill your employees to excel in the digital economy
Frequently asked questions
Access to lectures and assignments depends on your type of enrollment. If you take a course in audit mode, you will be able to see most course materials for free. To access graded assignments and to earn a Certificate, you will need to purchase the Certificate experience, during or after your audit. If you don't see the audit option:
The course may not offer an audit option. You can try a Free Trial instead, or apply for Financial Aid.
The course may offer 'Full Course, No Certificate' instead. This option lets you see all course materials, submit required assessments, and get a final grade. This also means that you will not be able to purchase a Certificate experience.
When you purchase a Certificate you get access to all course materials, including graded assignments. Upon completing the course, your electronic Certificate will be added to your Accomplishments page - from there, you can print your Certificate or add it to your LinkedIn profile. If you only want to read and view the course content, you can audit the course for free.
You will be eligible for a full refund until two weeks after your payment date, or (for courses that have just launched) until two weeks after the first session of the course begins, whichever is later. You cannot receive a refund once you’ve earned a Course Certificate, even if you complete the course within the two-week refund period. See our full refund policy.
More questions
Financial aid available,