This Machine Learning Capstone course uses various Python-based machine learning libraries, such as Pandas, sci-kit-learn, and Tensorflow/Keras. You will also learn to apply your machine-learning skills and demonstrate your proficiency in them. Before taking this course, you must complete all the previous courses in the IBM Machine Learning Professional Certificate.

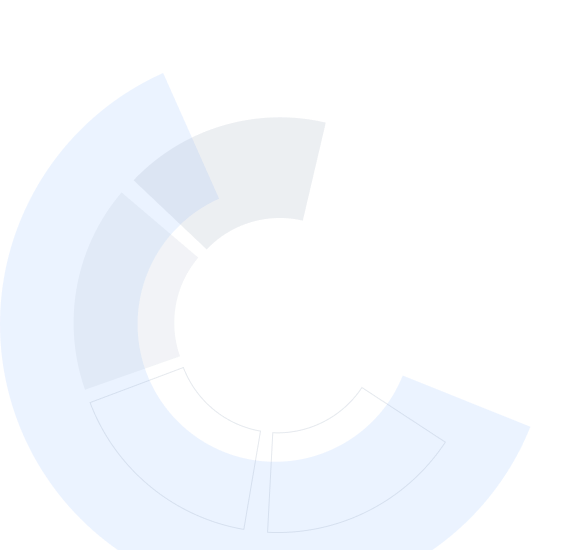
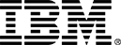
Machine Learning Capstone
This course is part of IBM Machine Learning Professional Certificate


Instructors: Yan Luo
15,408 already enrolled
Included with
(113 reviews)
Recommended experience
What you'll learn
Compare and contrast different machine learning algorithms by creating recommender systems in Python
Predict course ratings by training a neural network and constructing regression and classification models
Create recommendation systems by applying your knowledge of KNN, PCA, and non-negative matrix collaborative filtering
Develop a final presentation and evaluate your peers’ projects
Skills you'll gain
Details to know

Add to your LinkedIn profile
6 assignments
See how employees at top companies are mastering in-demand skills
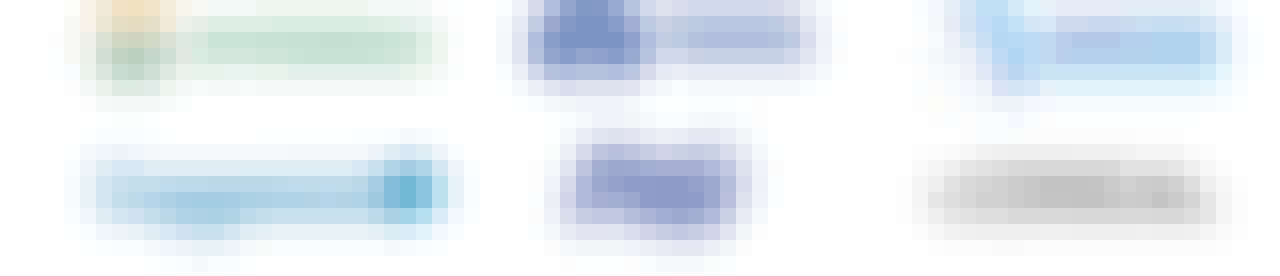
Build your Machine Learning expertise
- Learn new concepts from industry experts
- Gain a foundational understanding of a subject or tool
- Develop job-relevant skills with hands-on projects
- Earn a shareable career certificate from IBM
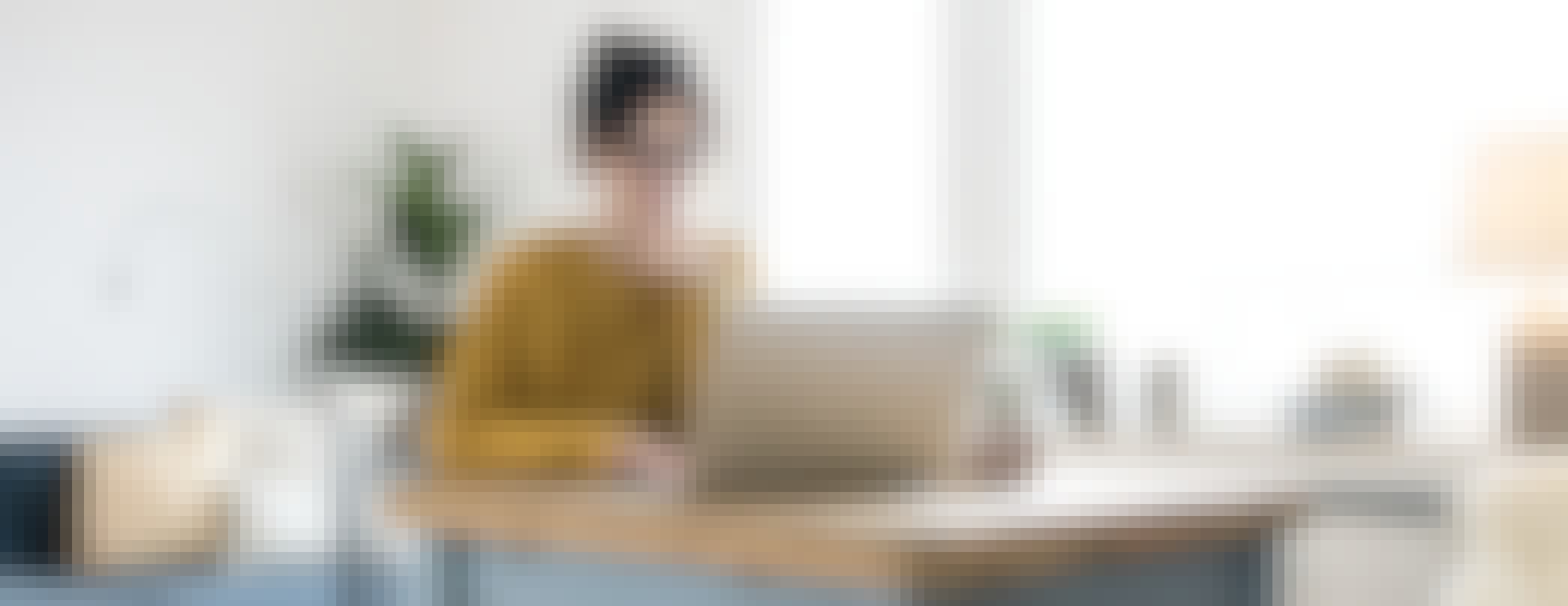
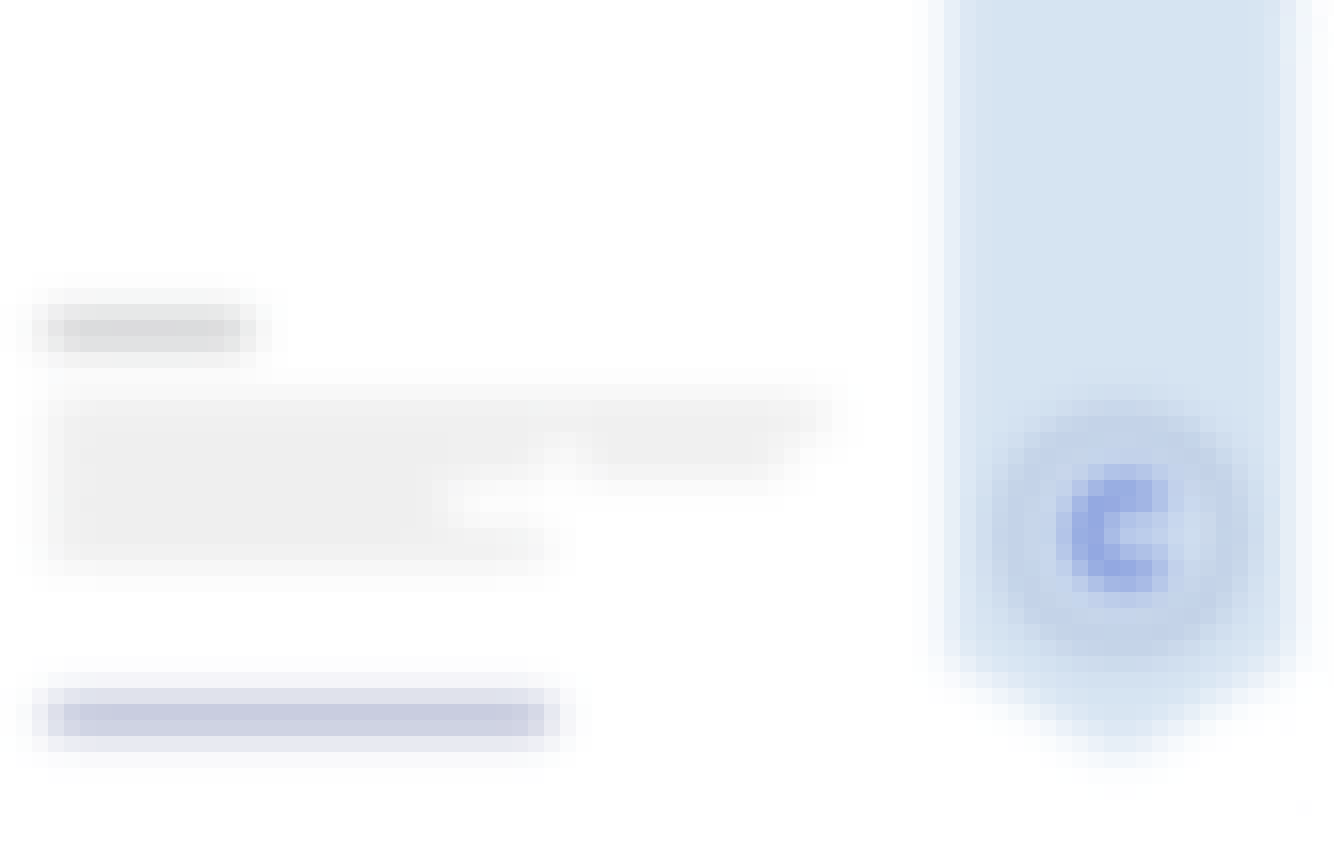
Earn a career certificate
Add this credential to your LinkedIn profile, resume, or CV
Share it on social media and in your performance review
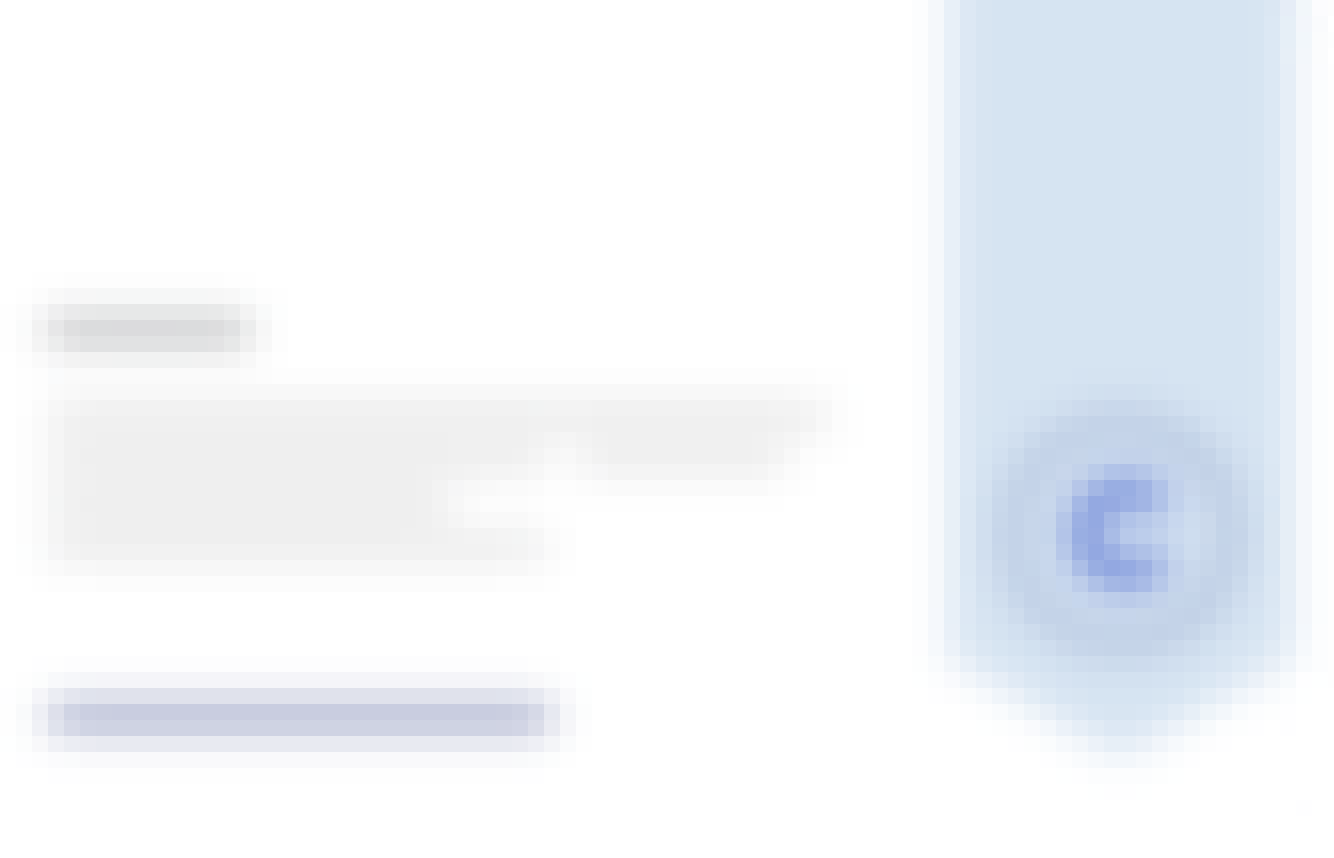
There are 5 modules in this course
In this module, you will be introduced to the idea of recommender systems. All labs in subsequent modules are based on this concept. You will also be provided with an overview of the capstone project. You will perform exploratory data analysis to find preliminary insights such as data patterns. You will also use it to check assumptions with the help of summary statistics and graphical representations of online course-related data sets such as course titles, course genres, and course enrollments. Next, you will extract a word-count vector called a “bag of words” (BoW) from course titles and descriptions. The BoW feature is probably the simplest but most effective feature characterizing textual data. It is widely used in many textual machine learning tasks. Finally, you will apply the cosine similarity measurement to calculate the course similarity using the extracted BoW feature vectors.
What's included
2 videos2 assignments3 app items5 plugins
In this module, you will create three course recommendation systems using different methods. In lab 1, you will create a course recommendation system based on user profile and course genre matrices by computing an interest score for each course and recommend the courses with the highest interest scores. In the second lab, you will generate a course similarity matrix to create the recommendation system. In the third lab, you will implement a clustering-based recommender system algorithm using K-means clustering and principal component analysis based on group members’ course enrollment history. In labs four and five you will use collaborative filtering to make predictions about a user’s interest based on a collection of other users’ similar preferences. In lab 4, you will perform KNN-based collaborative filtering and in lab 5, you will use non-negative matrix factorization.
What's included
1 video2 assignments3 app items2 plugins
In this module, you will predict course ratings using neural networks. In the first lab, you will train neural networks to predict course ratings while simultaneously extracting users' and items' latent features. In lab 2, you will be given course interaction feature vectors as input data. Using regression analysis, you will calculate numerical rating scores that predict whether a student will audit or complete a course. Lab 3 is similar to lab 2 but instead of using regression you will use a classification model. You will extract user and item embedding feature vectors from a neural network. With those embedding feature vectors, you will create an interaction feature vector and use that to build a classification model. The model maps the interaction feature vector to a rating mode that predicts whether a learner will audit or complete a course.
What's included
1 video2 assignments5 app items1 plugin
In this module, you will review guidelines and best practices for creating successful reports. As well you may wish to review instructions on creating PowerPoint presentations and how to save a PowerPoint as a PDF.
What's included
2 videos4 plugins
In this final module, you will be introduced to Streamlit and have the opportunity to build a Streamlit app to showcase your work in previous modules. You will complete your submission of screenshots from the hands-on labs for your peers to review. Once you have completed your submission you will then review the submission of one of your peers and grade their submission.
What's included
3 readings1 peer review4 plugins
Instructors


Offered by
Recommended if you're interested in Machine Learning
Howard University
University of Michigan
University of Alberta
University of Minnesota
Why people choose Coursera for their career




Learner reviews
113 reviews
- 5 stars
76.31%
- 4 stars
18.42%
- 3 stars
0.87%
- 2 stars
0.87%
- 1 star
3.50%
Showing 3 of 113
Reviewed on Aug 28, 2024
good for getting overview of different machine learning ways
Reviewed on Mar 23, 2024
It was really a quite informative and well planned course. Will continue to get more Professional Certificate from IBM related to ML-DL and Generative AI
New to Machine Learning? Start here.

Open new doors with Coursera Plus
Unlimited access to 10,000+ world-class courses, hands-on projects, and job-ready certificate programs - all included in your subscription
Advance your career with an online degree
Earn a degree from world-class universities - 100% online
Join over 3,400 global companies that choose Coursera for Business
Upskill your employees to excel in the digital economy
Frequently asked questions
To take this course you must have completed these five courses: Exploratory Data Analysis for Machine Learning, Supervised Machine Learning: Regression, Supervised Machine Learning: Classification, Unsupervised Machine Learning, Deep Learning and Reinforcement Learning .
Web browser, PowerPoint (optional), Text editor/IDE (optional), local Python runtime (optional)
Access to lectures and assignments depends on your type of enrollment. If you take a course in audit mode, you will be able to see most course materials for free. To access graded assignments and to earn a Certificate, you will need to purchase the Certificate experience, during or after your audit. If you don't see the audit option:
The course may not offer an audit option. You can try a Free Trial instead, or apply for Financial Aid.
The course may offer 'Full Course, No Certificate' instead. This option lets you see all course materials, submit required assessments, and get a final grade. This also means that you will not be able to purchase a Certificate experience.