This course aims to help you to ask better statistical questions when performing empirical research. We will discuss how to design informative studies, both when your predictions are correct, as when your predictions are wrong. We will question norms, and reflect on how we can improve research practices to ask more interesting questions. In practical hands on assignments you will learn techniques and tools that can be immediately implemented in your own research, such as thinking about the smallest effect size you are interested in, justifying your sample size, evaluate findings in the literature while keeping publication bias into account, performing a meta-analysis, and making your analyses computationally reproducible.

Cultivate your career with expert-led programs, job-ready certificates, and 10,000 ways to grow. All for $25/month, billed annually. Save now
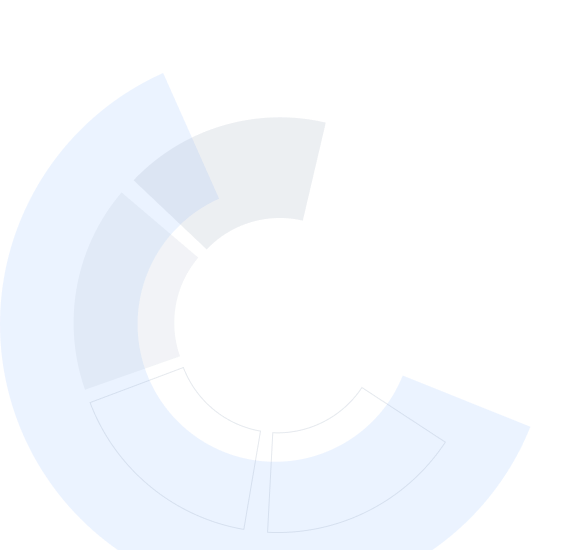
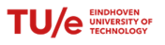
Improving Your Statistical Questions

Instructor: Daniel Lakens
Top Instructor
9,796 already enrolled
Included with
(111 reviews)
Recommended experience
What you'll learn
Ask better questions in empirical research
Design more informative studies
Evaluate the scientific literature taking bias into account
Reflect on current norms, and how you can improve your research practices
Skills you'll gain
Details to know

Add to your LinkedIn profile
12 assignments
See how employees at top companies are mastering in-demand skills
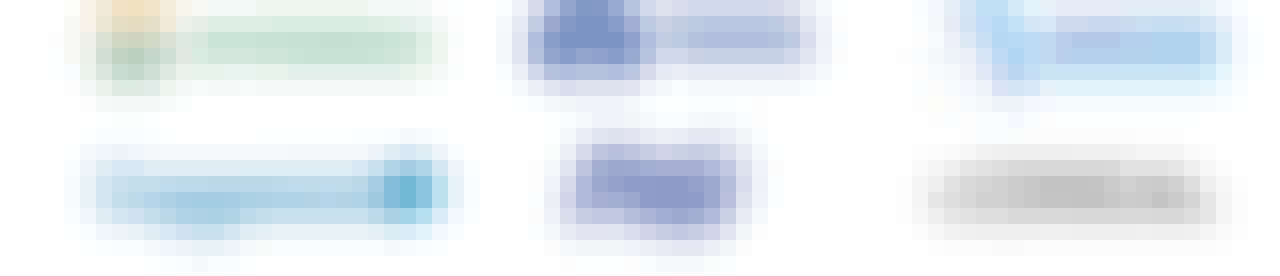
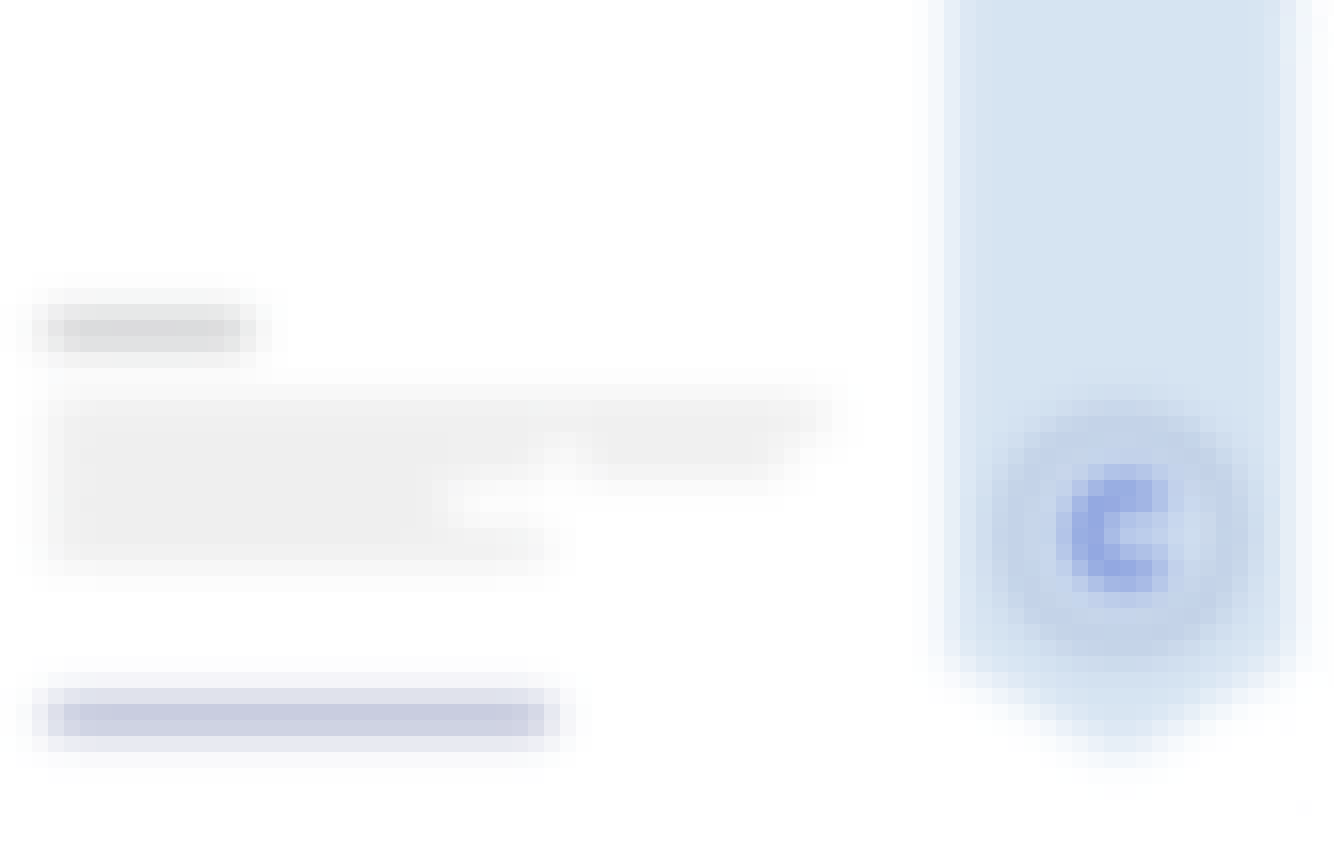
Earn a career certificate
Add this credential to your LinkedIn profile, resume, or CV
Share it on social media and in your performance review
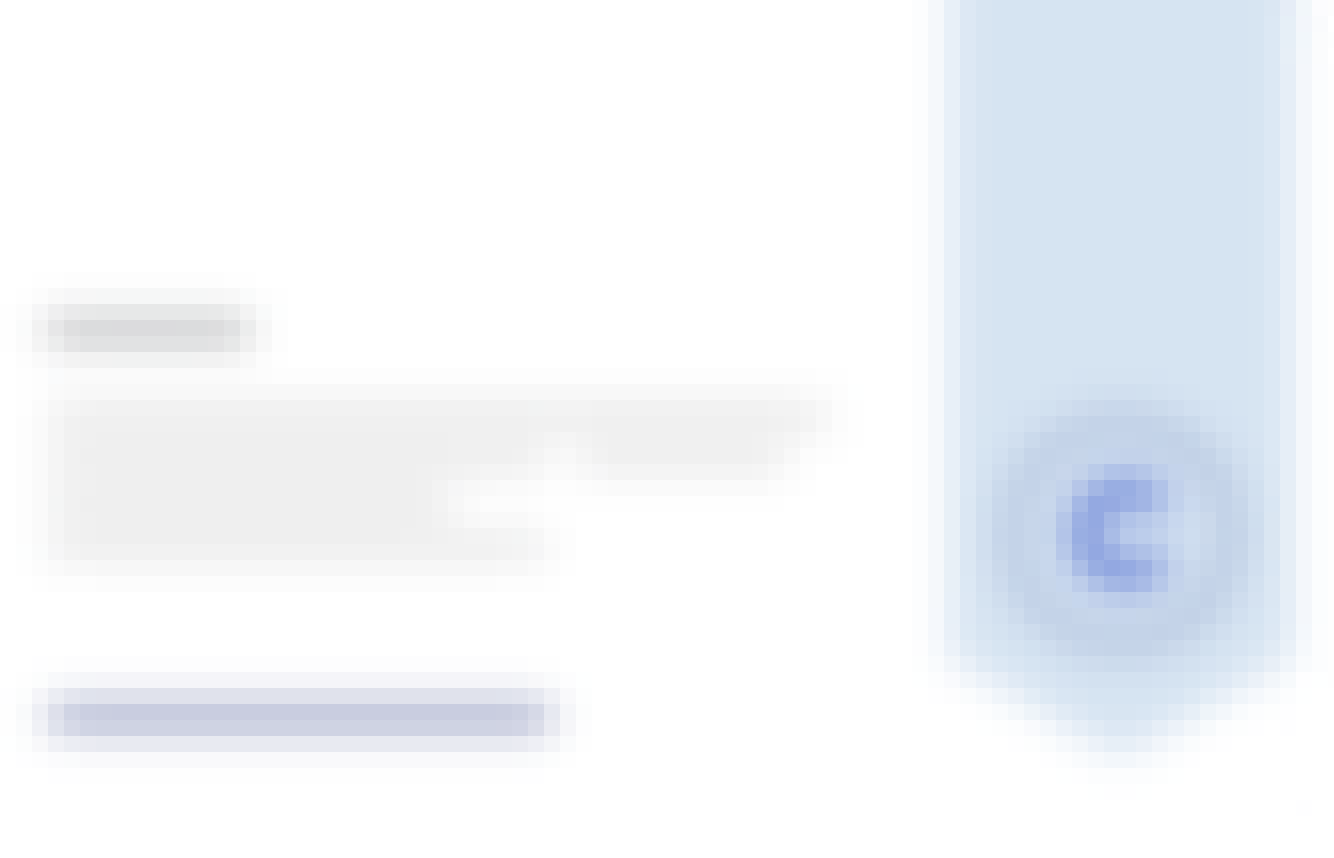
There are 6 modules in this course
One of the biggest improvements most researchers can make is to more clearly specify their statistical questions. When you perform a study, what is it you really want to know? What are different types of questions we can ask? Which question does a hypothesis test really answer, and is this answer actually what you are interested in, or is the question you are asking more about exploration, description, or prediction? How can we make riskier predictions than null-hypothesis tests, and why is this useful?
What's included
3 videos2 readings3 assignments
There is little use in making predictions if you can never be wrong - so how do we make sure your predictions are falsifiable? We discuss why falsifiable predictions are important, and how to make your predictions falsifiable in practice. One important aspect of making predictions falsifiable is to specify a range of values that is not predicted, and we will examine different approaches to specifying a smallest effect size of interest.
What's included
3 videos3 readings3 assignments
If studies are designed to answer a question, you should make sure the answer you will get after collecting data is informative. Instead of mindlessly setting Type 1 and Type 2 error rates, we will learn why it is important to be able to justify error rates, and some approaches how to do so. We discuss the benefits of using your smallest effect size of interest in power analyses, and why learning to simulate data is a useful tool. Simulations can help you to improve your understanding of statistics, enable you to design informative studies, and even ask novel questions.
What's included
3 videos2 readings2 assignments
Regrettably we work in a scientific enterprise where the published literature does not reflect real research. Publication bias and selection biases lead to a scientific literature that can’t be interpreted without taking these biases into account. We will discuss what real research lines look like, and how to meta-analytically evaluate the literature while keeping bias in mind.
What's included
3 videos4 readings3 assignments
We discuss three last topics. First, we will make sure other people can use your data to ask new questions, by making sure your data analysis is computationally reproducible. Then, we will reflect on how your philosophy of science influences the types of questions you will ask, and what you value as you do research. Finally, we discuss scientific integrity, and reflect on why our research practice is not always aligned with the best possible ways to provide reliable answers to scientific questions.
What's included
3 videos2 readings2 plugins
This module contains a graded exam. It covers content from the entire course. We recommend making this exam only after you went through all the other modules.
What's included
1 assignment
Instructor

Offered by
Recommended if you're interested in Probability and Statistics
Eindhoven University of Technology
University of Amsterdam
University of North Texas
Why people choose Coursera for their career




Learner reviews
111 reviews
- 5 stars
90.09%
- 4 stars
7.20%
- 3 stars
2.70%
- 2 stars
0%
- 1 star
0%
Showing 3 of 111
Reviewed on Apr 30, 2024
Excellent course and the teacher! Rich study materials and hand-on assignment. Good guide for research overall.
Reviewed on Dec 18, 2023
This was the best course that I have ever taken. Professor Lakens's excellent expression and wonderful lesson plan have created a thought-provoking review. I sincerely thank him
Reviewed on Oct 30, 2019
Daniel's second course as good as the first. He does a nice job!!

Open new doors with Coursera Plus
Unlimited access to 10,000+ world-class courses, hands-on projects, and job-ready certificate programs - all included in your subscription
Advance your career with an online degree
Earn a degree from world-class universities - 100% online
Join over 3,400 global companies that choose Coursera for Business
Upskill your employees to excel in the digital economy
Frequently asked questions
The course assumes basic knowledge about statistical inferences (t-tests, ANOVA) and some knowledge of designing research studies. The course is for intermediate level. Coursera offers basic introductions to statistics (which this course is not), and my previous MOOC 'Improving Your Statistical Inferences' might be a better starting point if you lack training in statistics. You do not need knowledge programming in R - we will use it as a fancy calculator by changing code (but not programming).
Access to lectures and assignments depends on your type of enrollment. If you take a course in audit mode, you will be able to see most course materials for free. To access graded assignments and to earn a Certificate, you will need to purchase the Certificate experience, during or after your audit. If you don't see the audit option:
The course may not offer an audit option. You can try a Free Trial instead, or apply for Financial Aid.
The course may offer 'Full Course, No Certificate' instead. This option lets you see all course materials, submit required assessments, and get a final grade. This also means that you will not be able to purchase a Certificate experience.
When you purchase a Certificate you get access to all course materials, including graded assignments. Upon completing the course, your electronic Certificate will be added to your Accomplishments page - from there, you can print your Certificate or add it to your LinkedIn profile. If you only want to read and view the course content, you can audit the course for free.
More questions
Financial aid available,