In this course, you’ll be learning about Computer Vision as a field of study and research. First we’ll be exploring several Computer Vision tasks and suggested approaches, from the classic Computer Vision perspective. Then we’ll introduce Deep Learning methods and apply them to some of the same problems. We will analyze the results and discuss advantages and drawbacks of both types of methods. We'll use tutorials to let you explore hands-on some of the modern machine learning tools and software libraries. Examples of Computer Vision tasks where Deep Learning can be applied include: image classification, image classification with localization, object detection, object segmentation, facial recognition, and activity or pose estimation.

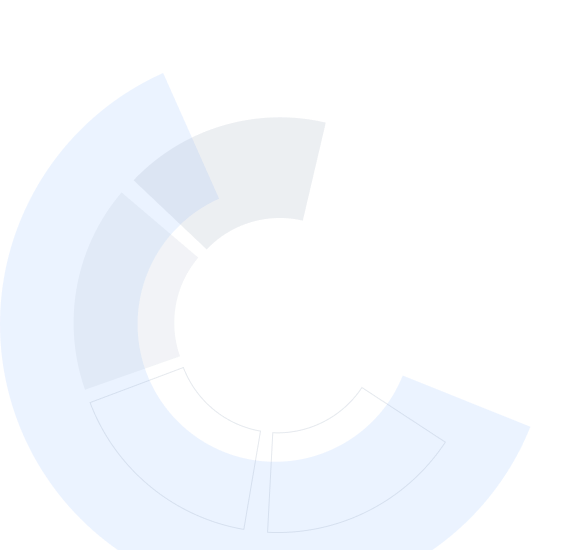
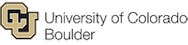
Deep Learning Applications for Computer Vision

Instructor: Ioana Fleming
8,355 already enrolled
Included with
(88 reviews)
Recommended experience
What you'll learn
Learners will be able to explain what Computer Vision is and give examples of Computer Vision tasks.
Learners will be able to describe the process behind classic algorithmic solutions to Computer Vision tasks and explain their pros and cons.
Learners will be able to use hands-on modern machine learning tools and python libraries.
Skills you'll gain
Details to know

Add to your LinkedIn profile
4 assignments
See how employees at top companies are mastering in-demand skills
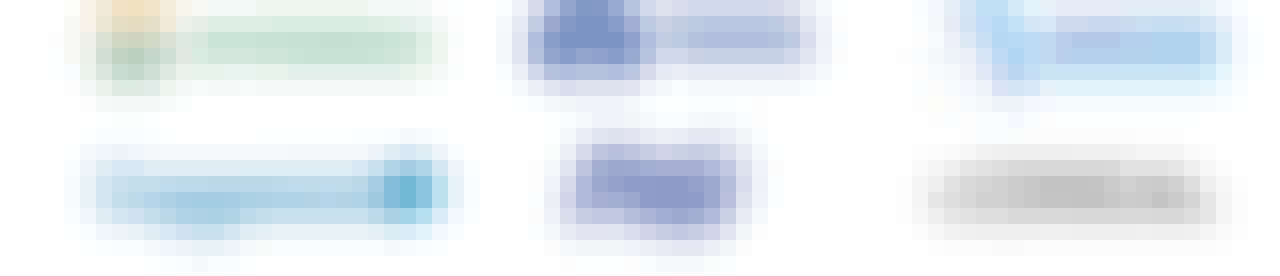
There are 5 modules in this course
In this module, you will learn about the field of Computer Vision. Computer Vision has the goal of extracting information from images. We will go over the major categories of tasks of Computer Vision and we will give examples of applications from each category. With the adoption of Machine Learning and Deep Learning techniques, we will look at how this has impacted the field of Computer Vision.
What's included
4 videos13 readings1 assignment1 discussion prompt
In this module, you will learn about classic Computer Vision tools and techniques. We will explore the convolution operation, linear filters, and algorithms for detecting image features.
What's included
5 videos10 readings1 assignment
In this module we will first review the challenges for object recognition in Classic Computer Vision. Then we will go through the steps of achieving object recognition and image classification in the Classic Computer Vision pipeline.
What's included
3 videos2 readings1 assignment
In this module we will compare how the image classification pipeline with neural networks differs than the one with classic computer vision tools. Then we will review the basic components of a neural network. We will conclude with a tutorial in Tensor flow where we will practice how to build, train and use a neural network for image classification predictions.
What's included
4 videos5 readings1 peer review1 ungraded lab
In this module we will learn about the components of Convolutional Neural Networks. We will study the parameters and hyperparameters that describe a deep network and explore their role in improving the accuracy of the deep learning models. We will conclude with a tutorial in Tensor Flow where we will practice building, training and using a deep neural network for image classification.
What's included
6 videos10 readings1 assignment1 peer review1 ungraded lab
Earn a career certificate
Add this credential to your LinkedIn profile, resume, or CV. Share it on social media and in your performance review.
Build toward a degree
This course is part of the following degree program(s) offered by University of Colorado Boulder. If you are admitted and enroll, your completed coursework may count toward your degree learning and your progress can transfer with you.¹
Instructor

Offered by
Explore more from Machine Learning
- Status: Free Trial
MathWorks
University of Colorado Boulder
- Status: Free Trial
Edge Impulse
Why people choose Coursera for their career




Learner reviews
88 reviews
- 5 stars
76.13%
- 4 stars
18.18%
- 3 stars
3.40%
- 2 stars
0%
- 1 star
2.27%
Showing 3 of 88
Reviewed on Jun 16, 2022
Learnt many things and most exciting was Python code part
Reviewed on Aug 24, 2024
Great content and clear, succinct explanations of the concepts!
Reviewed on Jan 12, 2025
A well comprehensive course with good explainations for terminologies and concepts in DL

Open new doors with Coursera Plus
Unlimited access to 10,000+ world-class courses, hands-on projects, and job-ready certificate programs - all included in your subscription
Advance your career with an online degree
Earn a degree from world-class universities - 100% online
Join over 3,400 global companies that choose Coursera for Business
Upskill your employees to excel in the digital economy
Frequently asked questions
A cross-listed course is offered under two or more CU Boulder degree programs on Coursera. For example, Dynamic Programming, Greedy Algorithms is offered as both CSCA 5414 for the MS-CS and DTSA 5503 for the MS-DS.
· You may not earn credit for more than one version of a cross-listed course.
· You can identify cross-listed courses by checking your program’s student handbook.
· Your transcript will be affected. Cross-listed courses are considered equivalent when evaluating graduation requirements. However, we encourage you to take your program's versions of cross-listed courses (when available) to ensure your CU transcript reflects the substantial amount of coursework you are completing directly in your home department. Any courses you complete from another program will appear on your CU transcript with that program’s course prefix (e.g., DTSA vs. CSCA).
· Programs may have different minimum grade requirements for admission and graduation. For example, the MS-DS requires a C or better on all courses for graduation (and a 3.0 pathway GPA for admission), whereas the MS-CS requires a B or better on all breadth courses and a C or better on all elective courses for graduation (and a B or better on each pathway course for admission). All programs require students to maintain a 3.0 cumulative GPA for admission and graduation.
Yes. Cross-listed courses are considered equivalent when evaluating graduation requirements. You can identify cross-listed courses by checking your program’s student handbook.
You may upgrade and pay tuition during any open enrollment period to earn graduate-level CU Boulder credit for << this course/ courses in this specialization>>. Because << this course is / these courses are >> cross listed in both the MS in Computer Science and the MS in Data Science programs, you will need to determine which program you would like to earn the credit from before you upgrade.
MS in Data Science (MS-DS) Credit: To upgrade to the for-credit data science (DTSA) version of << this course / these courses >>, use the MS-DS enrollment form. See How It Works.
MS in Computer Science (MS-CS) Credit: To upgrade to the for-credit computer science (CSCA) version of << this course / these courses >>, use the MS-CS enrollment form. See How It Works.
If you are unsure of which program is the best fit for you, review the MS-CS and MS-DS program websites, and then contact datascience@colorado.edu or mscscoursera-info@colorado.edu if you still have questions.
More questions
Financial aid available,