This course introduces the key steps involved in the data mining pipeline, including data understanding, data preprocessing, data warehousing, data modeling, interpretation and evaluation, and real-world applications.

Heat up your career with 40% off top courses from Google, Adobe, and more. Save today.
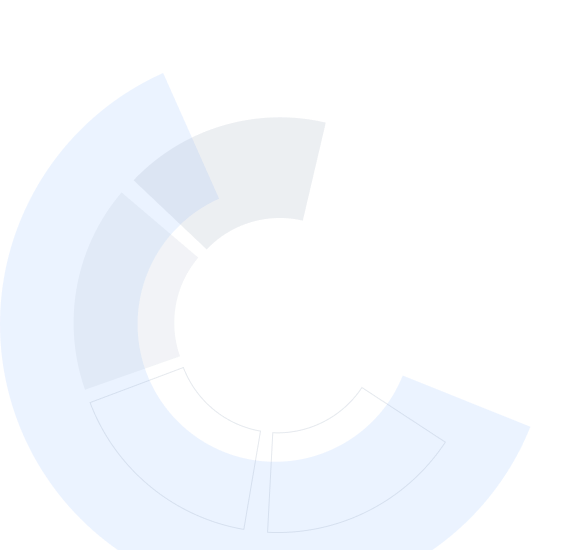
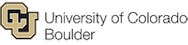
Data Mining Pipeline
This course is part of Data Mining Foundations and Practice Specialization

Instructor: Qin (Christine) Lv
10,104 already enrolled
Included with
(92 reviews)
Recommended experience
What you'll learn
Identify the key components of the data mining pipeline and describe how they're related.
Identify particular challenges presented by each component of the data mining pipeline.
Apply techniques to address challenges in each component of the data mining pipeline.
Skills you'll gain
Details to know

Add to your LinkedIn profile
See how employees at top companies are mastering in-demand skills
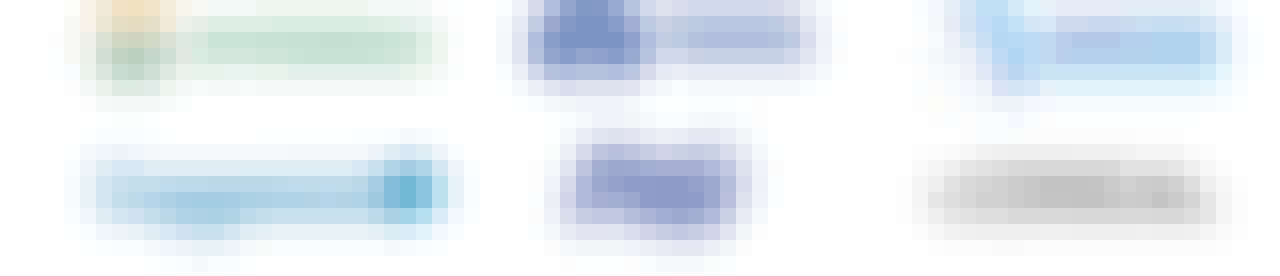
Build your subject-matter expertise
- Learn new concepts from industry experts
- Gain a foundational understanding of a subject or tool
- Develop job-relevant skills with hands-on projects
- Earn a shareable career certificate
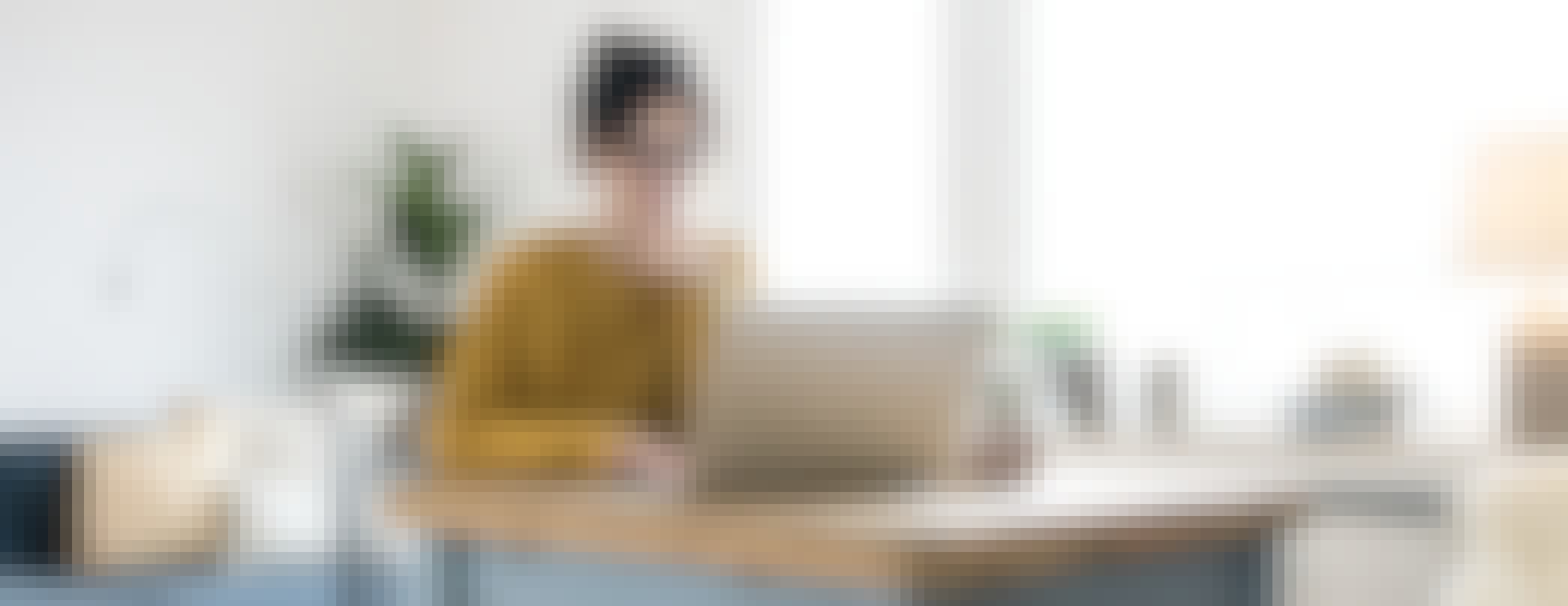
There are 4 modules in this course
This week provides you with an introduction to the Data Mining Specialization and this course, Data Mining Pipeline. As you begin, you will get introduced to the four views of data mining and the key components in the data mining pipeline.
What's included
8 videos3 readings2 peer reviews1 discussion prompt
This week covers data understanding by identifying key data properties and applying techniques to characterize different datasets.
What's included
6 videos1 programming assignment
This week explains why data preprocessing is needed and what techniques can be used to preprocess data.
What's included
6 videos1 programming assignment
This week covers the key characteristics of data warehousing and the techniques to support data warehousing.
What's included
4 videos1 programming assignment
Earn a career certificate
Add this credential to your LinkedIn profile, resume, or CV. Share it on social media and in your performance review.
Build toward a degree
This course is part of the following degree program(s) offered by University of Colorado Boulder. If you are admitted and enroll, your completed coursework may count toward your degree learning and your progress can transfer with you.¹
Instructor

Offered by
Explore more from Data Analysis
- Status: Free Trial
University of Colorado Boulder
- Status: Free Trial
University of Colorado Boulder
- Status: Free Trial
University of Illinois Urbana-Champaign
- Status: Free
Coursera Project Network
Why people choose Coursera for their career




Learner reviews
92 reviews
- 5 stars
60.86%
- 4 stars
9.78%
- 3 stars
5.43%
- 2 stars
5.43%
- 1 star
18.47%
Showing 3 of 92
Reviewed on Oct 1, 2023
This course was recently updated. I feel it's much better than the prior version. The videos are easier to follow, and the assignments are cleaned up as well.

Open new doors with Coursera Plus
Unlimited access to 10,000+ world-class courses, hands-on projects, and job-ready certificate programs - all included in your subscription
Advance your career with an online degree
Earn a degree from world-class universities - 100% online
Join over 3,400 global companies that choose Coursera for Business
Upskill your employees to excel in the digital economy
Frequently asked questions
A cross-listed course is offered under two or more CU Boulder degree programs on Coursera. For example, Dynamic Programming, Greedy Algorithms is offered as both CSCA 5414 for the MS-CS and DTSA 5503 for the MS-DS.
· You may not earn credit for more than one version of a cross-listed course.
· You can identify cross-listed courses by checking your program’s student handbook.
· Your transcript will be affected. Cross-listed courses are considered equivalent when evaluating graduation requirements. However, we encourage you to take your program's versions of cross-listed courses (when available) to ensure your CU transcript reflects the substantial amount of coursework you are completing directly in your home department. Any courses you complete from another program will appear on your CU transcript with that program’s course prefix (e.g., DTSA vs. CSCA).
· Programs may have different minimum grade requirements for admission and graduation. For example, the MS-DS requires a C or better on all courses for graduation (and a 3.0 pathway GPA for admission), whereas the MS-CS requires a B or better on all breadth courses and a C or better on all elective courses for graduation (and a B or better on each pathway course for admission). All programs require students to maintain a 3.0 cumulative GPA for admission and graduation.
Yes. Cross-listed courses are considered equivalent when evaluating graduation requirements. You can identify cross-listed courses by checking your program’s student handbook.
You may upgrade and pay tuition during any open enrollment period to earn graduate-level CU Boulder credit for << this course/ courses in this specialization>>. Because << this course is / these courses are >> cross listed in both the MS in Computer Science and the MS in Data Science programs, you will need to determine which program you would like to earn the credit from before you upgrade.
MS in Data Science (MS-DS) Credit: To upgrade to the for-credit data science (DTSA) version of << this course / these courses >>, use the MS-DS enrollment form. See How It Works.
MS in Computer Science (MS-CS) Credit: To upgrade to the for-credit computer science (CSCA) version of << this course / these courses >>, use the MS-CS enrollment form. See How It Works.
If you are unsure of which program is the best fit for you, review the MS-CS and MS-DS program websites, and then contact datascience@colorado.edu or mscscoursera-info@colorado.edu if you still have questions.
More questions
Financial aid available,