If you are a software developer who wants to build scalable AI-powered algorithms, you need to understand how to use the tools to build them. This course is part of the DeepLearning.AI TensorFlow Developer Specialization and will teach you best practices for using TensorFlow, a popular open-source framework for machine learning.

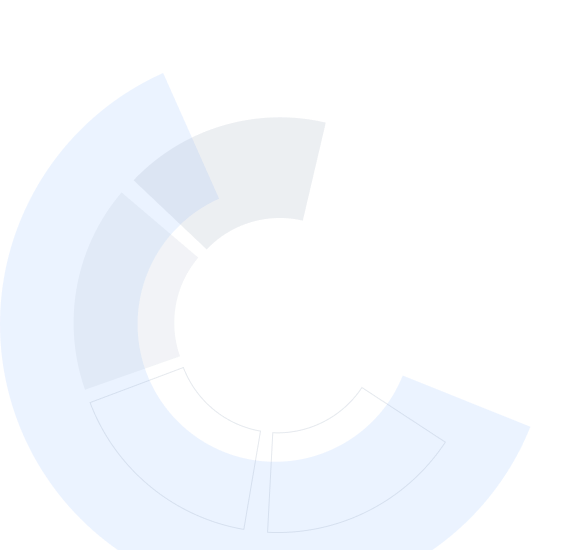
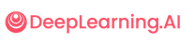
Convolutional Neural Networks in TensorFlow
This course is part of DeepLearning.AI TensorFlow Developer Professional Certificate

Instructor: Laurence Moroney
154,309 already enrolled
(8,150 reviews)
Recommended experience
What you'll learn
Handle real-world image data
Plot loss and accuracy
Explore strategies to prevent overfitting, including augmentation and dropout
Learn transfer learning and how learned features can be extracted from models
Skills you'll gain
Details to know

Add to your LinkedIn profile
4 assignments
See how employees at top companies are mastering in-demand skills
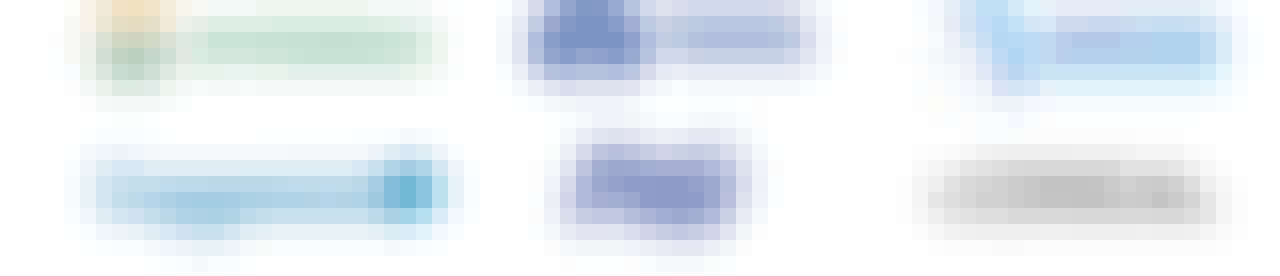
Build your Machine Learning expertise
- Learn new concepts from industry experts
- Gain a foundational understanding of a subject or tool
- Develop job-relevant skills with hands-on projects
- Earn a shareable career certificate from DeepLearning.AI
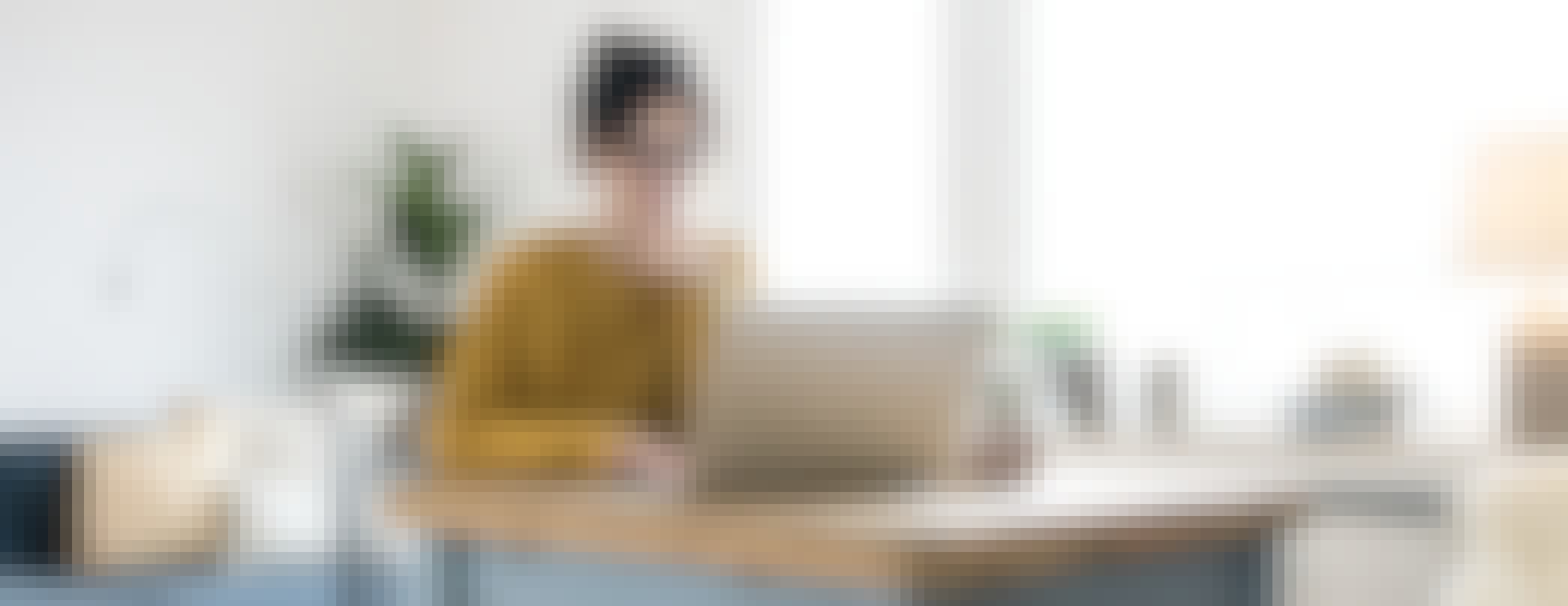
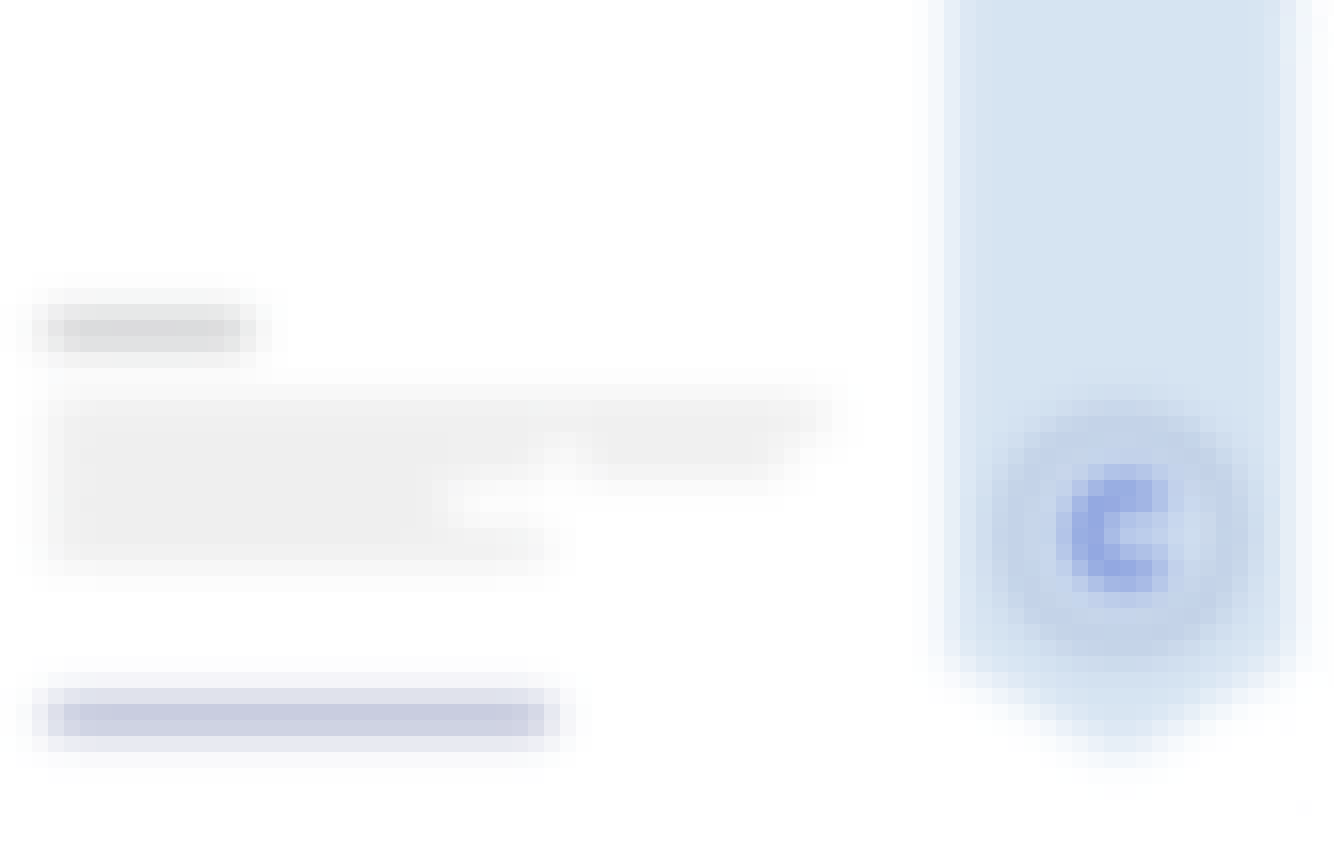
Earn a career certificate
Add this credential to your LinkedIn profile, resume, or CV
Share it on social media and in your performance review
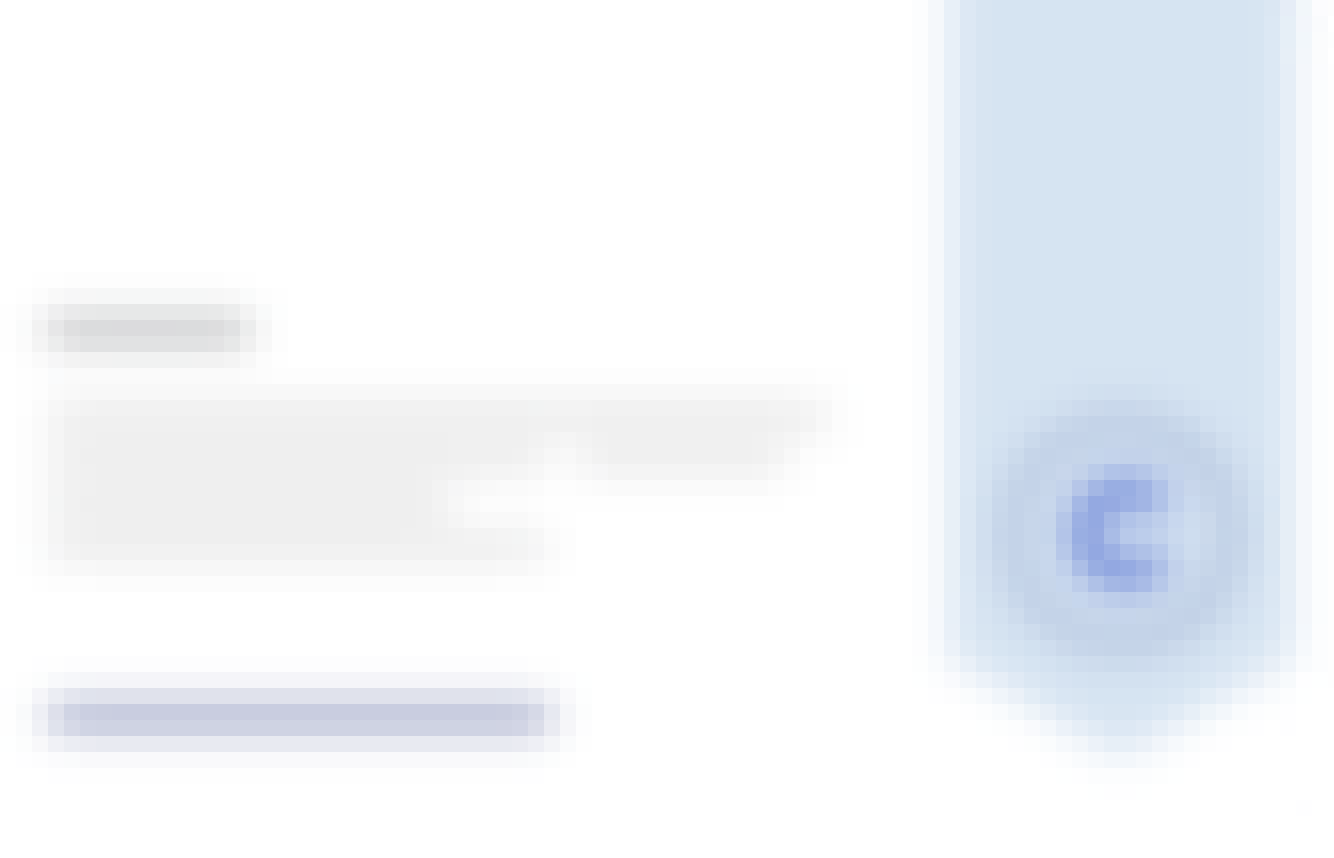
There are 4 modules in this course
In the first course in this specialization, you had an introduction to TensorFlow, and how, with its high level APIs you could do basic image classification, and you learned a little bit about Convolutional Neural Networks (ConvNets). In this course you'll go deeper into using ConvNets with real-world data, and learn about techniques that you can use to improve your ConvNet performance, particularly when doing image classification! In Week 1, this week, you'll get started by looking at a much larger dataset than you've been using thus far: The Cats and Dogs dataset which had been a Kaggle Challenge in image classification!
What's included
8 videos8 readings1 assignment1 programming assignment1 ungraded lab
You've heard the term overfitting a number of times to this point. Overfitting is simply the concept of being over specialized in training -- namely that your model is very good at classifying what it is trained for, but not so good at classifying things that it hasn't seen. In order to generalize your model more effectively, you will of course need a greater breadth of samples to train it on. That's not always possible, but a nice potential shortcut to this is Image Augmentation, where you tweak the training set to potentially increase the diversity of subjects it covers. You'll learn all about that this week!
What's included
7 videos4 readings1 assignment1 programming assignment2 ungraded labs
Building models for yourself is great, and can be very powerful. But, as you've seen, you can be limited by the data you have on hand. Not everybody has access to massive datasets or the compute power that's needed to train them effectively. Transfer learning can help solve this -- where people with models trained on large datasets train them, so that you can either use them directly, or, you can use the features that they have learned and apply them to your scenario. This is Transfer learning, and you'll look into that this week!
What's included
7 videos4 readings1 assignment1 programming assignment1 ungraded lab
You've come a long way, Congratulations! One more thing to do before we move off of ConvNets to the next module, and that's to go beyond binary classification. Each of the examples you've done so far involved classifying one thing or another -- horse or human, cat or dog. When moving beyond binary into Categorical classification there are some coding considerations you need to take into account. You'll look at them this week!
What's included
6 videos7 readings1 assignment1 programming assignment1 ungraded lab
Instructor

Offered by
Recommended if you're interested in Machine Learning
Coursera Project Network
DeepLearning.AI
DeepLearning.AI
Johns Hopkins University
Why people choose Coursera for their career




Learner reviews
Showing 3 of 8150
8,150 reviews
- 5 stars
79.25%
- 4 stars
15.48%
- 3 stars
3.48%
- 2 stars
0.99%
- 1 star
0.78%
New to Machine Learning? Start here.

Open new doors with Coursera Plus
Unlimited access to 7,000+ world-class courses, hands-on projects, and job-ready certificate programs - all included in your subscription
Advance your career with an online degree
Earn a degree from world-class universities - 100% online
Join over 3,400 global companies that choose Coursera for Business
Upskill your employees to excel in the digital economy
Frequently asked questions
Access to lectures and assignments depends on your type of enrollment. If you take a course in audit mode, you will be able to see most course materials for free. To access graded assignments and to earn a Certificate, you will need to purchase the Certificate experience, during or after your audit. If you don't see the audit option:
The course may not offer an audit option. You can try a Free Trial instead, or apply for Financial Aid.
The course may offer 'Full Course, No Certificate' instead. This option lets you see all course materials, submit required assessments, and get a final grade. This also means that you will not be able to purchase a Certificate experience.
When you enroll in the course, you get access to all of the courses in the Certificate, and you earn a certificate when you complete the work. Your electronic Certificate will be added to your Accomplishments page - from there, you can print your Certificate or add it to your LinkedIn profile. If you only want to read and view the course content, you can audit the course for free.
If you subscribed, you get a 7-day free trial during which you can cancel at no penalty. After that, we don’t give refunds, but you can cancel your subscription at any time. See our full refund policy.