In this final course, you will put together your knowledge from Courses 1, 2 and 3 to implement a complete RL solution to a problem. This capstone will let you see how each component---problem formulation, algorithm selection, parameter selection and representation design---fits together into a complete solution, and how to make appropriate choices when deploying RL in the real world. This project will require you to implement both the environment to stimulate your problem, and a control agent with Neural Network function approximation. In addition, you will conduct a scientific study of your learning system to develop your ability to assess the robustness of RL agents. To use RL in the real world, it is critical to (a) appropriately formalize the problem as an MDP, (b) select appropriate algorithms, (c ) identify what choices in your implementation will have large impacts on performance and (d) validate the expected behaviour of your algorithms. This capstone is valuable for anyone who is planning on using RL to solve real problems.

Early bird sale! Unlock 10,000+ courses from Google, IBM, and more for 50% off. Save today.
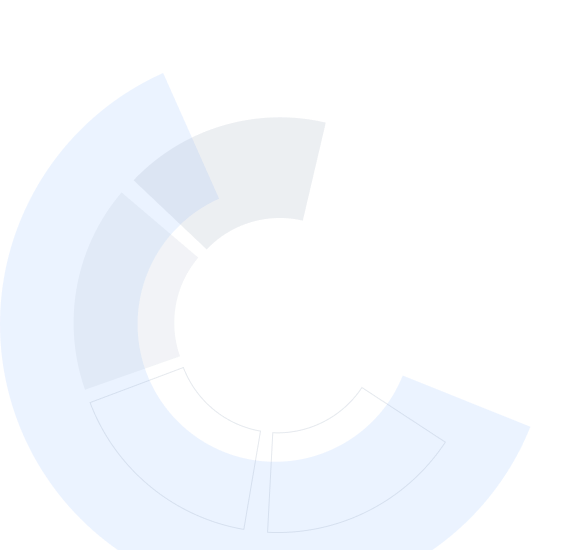
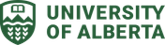
A Complete Reinforcement Learning System (Capstone)
This course is part of Reinforcement Learning Specialization


Instructors: Martha White
23,343 already enrolled
Included with
(641 reviews)
Recommended experience
Skills you'll gain
Details to know

Add to your LinkedIn profile
2 assignments
See how employees at top companies are mastering in-demand skills
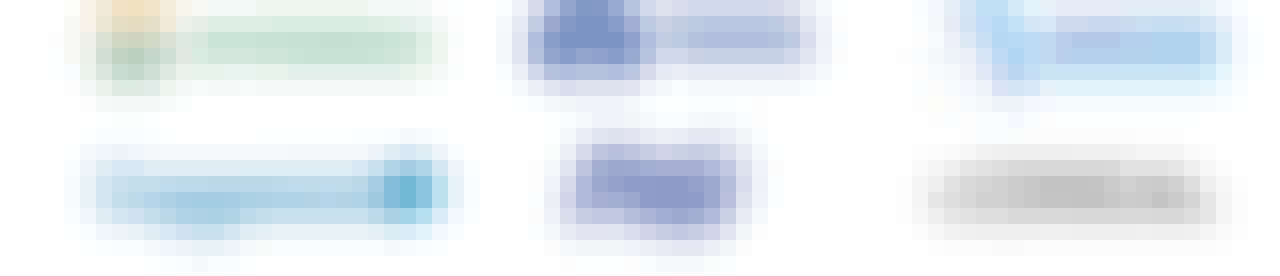
Build your subject-matter expertise
- Learn new concepts from industry experts
- Gain a foundational understanding of a subject or tool
- Develop job-relevant skills with hands-on projects
- Earn a shareable career certificate
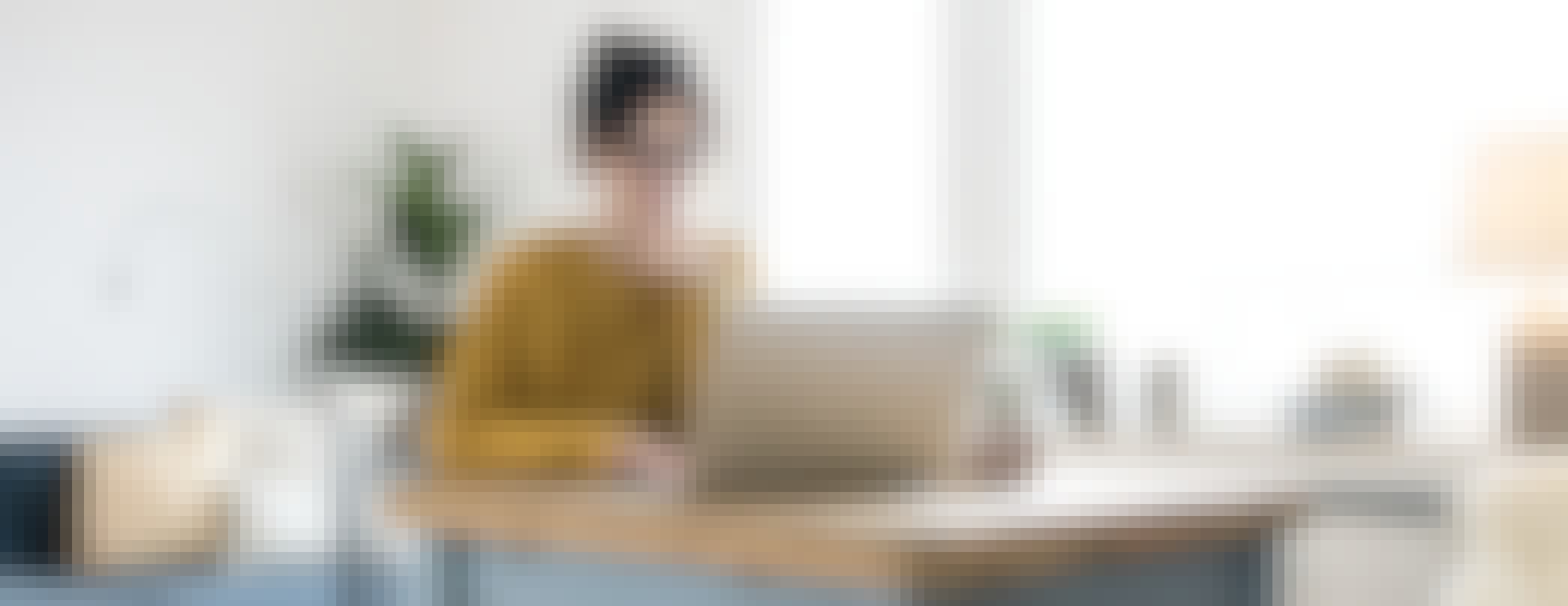
There are 6 modules in this course
Welcome to the final capstone course of the Reinforcement Learning Specialization!!
What's included
2 videos2 readings1 discussion prompt
This week you will read a description of a problem, and translate it into an MDP. You will complete skeleton code for this environment, to obtain a complete MDP for use in this capstone project.
What's included
4 videos1 programming assignment
This week you will select from three algorithms, to learn a policy for the environment. You will reflect on and discuss the appropriateness of each algorithm for this environment.
What's included
7 videos1 assignment
This week you will identify key parameters that affect the performance of your agent. The goal is to understand the space of options, to later enable you to choose which parameter you will investigate in-depth for your agent.
What's included
4 videos1 assignment
This week, you will implement your agent using Expected Sarsa or Q-learning with RMSProp and Neural Networks. To use NNs, you will have to use a more careful stepsize selection strategy, which is why you will use RMSProp. You will also verify the correctness of your agent.
What's included
6 videos1 programming assignment
This week you will identify a parameter to study, for your agent. Once you select the parameter to study, we will provide you with a range of values and specific values for other parameters. You will write a script to run your agent and environment on the set of parameters, to determine performance across these parameters. You will gain insight into the impact of parameters on agent performance. You will also get to visualize the agents that you learn. Your parameter study will consist of an array of values that we will check for correctness.
What's included
6 videos1 programming assignment
Earn a career certificate
Add this credential to your LinkedIn profile, resume, or CV. Share it on social media and in your performance review.
Instructors


Explore more from Machine Learning
- Status: Free Trial
University of Alberta
- Status: Free Trial
- Status: Free Trial
University of Alberta
- Status: Free Trial
Why people choose Coursera for their career




Learner reviews
641 reviews
- 5 stars
78%
- 4 stars
15.91%
- 3 stars
4.99%
- 2 stars
0.62%
- 1 star
0.46%
Showing 3 of 641
Reviewed on Jan 12, 2022
It may have been useful to provide less guidance to the students to make sure they develop the required skills.
Reviewed on Jul 10, 2020
Strongly recommend this course to others. The project could be a little more challenging though. Thanks, Martha, Adam, and RAs, for your good teaching!
Reviewed on Aug 2, 2020
One of the most amazing set of courses that I have ever been through. This neither makes the stuff look difficult nor does it compromise on quality, absolutely the best.

Open new doors with Coursera Plus
Unlimited access to 10,000+ world-class courses, hands-on projects, and job-ready certificate programs - all included in your subscription
Advance your career with an online degree
Earn a degree from world-class universities - 100% online
Join over 3,400 global companies that choose Coursera for Business
Upskill your employees to excel in the digital economy
Frequently asked questions
Access to lectures and assignments depends on your type of enrollment. If you take a course in audit mode, you will be able to see most course materials for free. To access graded assignments and to earn a Certificate, you will need to purchase the Certificate experience, during or after your audit. If you don't see the audit option:
The course may not offer an audit option. You can try a Free Trial instead, or apply for Financial Aid.
The course may offer 'Full Course, No Certificate' instead. This option lets you see all course materials, submit required assessments, and get a final grade. This also means that you will not be able to purchase a Certificate experience.
When you enroll in the course, you get access to all of the courses in the Specialization, and you earn a certificate when you complete the work. Your electronic Certificate will be added to your Accomplishments page - from there, you can print your Certificate or add it to your LinkedIn profile. If you only want to read and view the course content, you can audit the course for free.
If you subscribed, you get a 7-day free trial during which you can cancel at no penalty. After that, we don’t give refunds, but you can cancel your subscription at any time. See our full refund policy.
More questions
Financial aid available,