Machine learning systems used in Clinical Decision Support Systems (CDSS) require further external validation, calibration analysis, assessment of bias and fairness. In this course, the main concepts of machine learning evaluation adopted in CDSS will be explained. Furthermore, decision curve analysis along with human-centred CDSS that need to be explainable will be discussed. Finally, privacy concerns of deep learning models and potential adversarial attacks will be presented along with the vision for a new generation of explainable and privacy-preserved CDSS.

Heat up your career with 40% off top courses from Google, Adobe, and more. Save today.
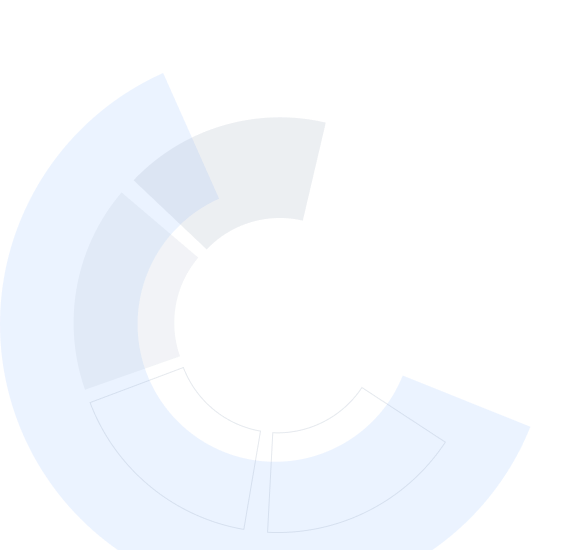
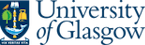
Clinical Decision Support Systems - CDSS 4
This course is part of Informed Clinical Decision Making using Deep Learning Specialization

Instructor: Fani Deligianni
Included with
What you'll learn
Evaluating Clinical Decision Support Systems
Bias, Calibration and Fairness in Machine Learning Models
Decision Curve Analysis and Human-Centred Clinical Decision Support Systems
Privacy concerns in Clinical Decision Support Systems
Skills you'll gain
Details to know

Add to your LinkedIn profile
5 assignments
See how employees at top companies are mastering in-demand skills
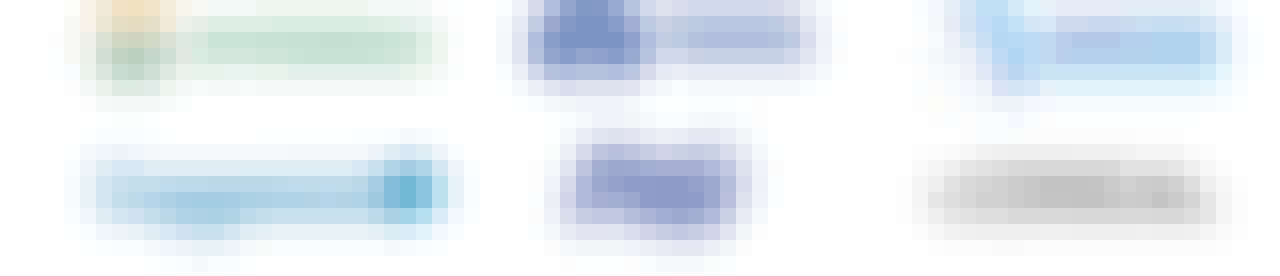
Build your subject-matter expertise
- Learn new concepts from industry experts
- Gain a foundational understanding of a subject or tool
- Develop job-relevant skills with hands-on projects
- Earn a shareable career certificate
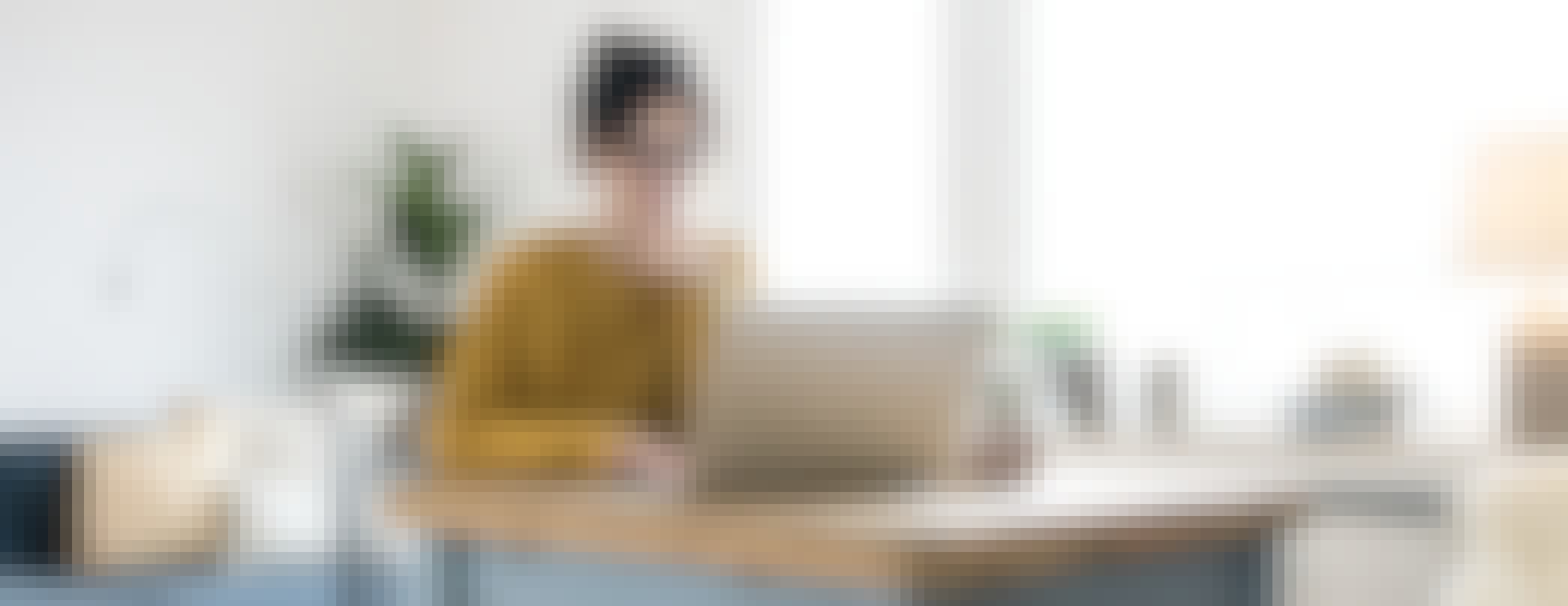
There are 4 modules in this course
Adopting a machine learning model in a Clinical Decision Support System (CDSS) requires several steps that involve external validation, bias assessment and calibration, 'fairness' assessment, clinical usefulness, ability to explain the model's decision and privacy-aware machine learning models. In this module, we are going to discuss these concepts and provide several examples from state-of-the-art research in the area. External validation and bias assessment have become the norm in clinical prediction models. Further work is required to assess and adopt deep learning models under these conditions. On the other hand, research in 'fairness', human-centred CDSS and privacy concerns of machine learning models are areas of active research. The first week is going to cover the ground around the difference between reproducibility and generalisability. Furthermore, calibration assessment in clinical prediction models will be explored while how different deep learning architectures affect calibration will be discussed.
What's included
4 videos3 readings1 assignment1 discussion prompt
Naively, machine learning can be thought as a way to come to decisions that are free from prejudice and social biases. However, recent evidence show how machine learning models learn from biases in historic data and reproduce unfair decisions in similar ways. Detecting biases against subgroups in machine learning models is challenging also due to the fact that these models have not been designed or trained to discriminate deliberately. Defining 'fairness' metrics and investigating ways in ensuring that minority groups are not disadvantaged from machine learning models' decisions is an active research area.
What's included
3 videos3 readings1 assignment1 discussion prompt
Decision curve analysis is used to assess clinical usefulness of a prediction model by estimating the net benefit with is a trade-off of the precision and accuracy of the model. Based on this approach the strategy of ‘intervention for all’ and ‘intervention for none’ is compared to the model’s net benefit. Decision curve analysis is a human-centred approach of assessing clinical usefulness, since it requires experts’ opinion. Ethical Artificial Intelligence initiative indicate that a human-centred approach in clinical decision support systems is required to enable accountability, safety and oversight while the ensure ‘fairness’ and transparency.
What's included
3 videos3 readings1 assignment1 discussion prompt
Deep learning models have remarkable ability to memorise data even when they do not overfit. In other words, the models themselves can expose information about the patients that compromise their privacy. This can results in unintentional data leakage in inference and also provide opportunities for malicious attacks. We will overview common privacy attacks and defences against them. Finally, we will discuss adversarial attacks against deep learning explanations.
What's included
3 videos3 readings2 assignments1 discussion prompt
Earn a career certificate
Add this credential to your LinkedIn profile, resume, or CV. Share it on social media and in your performance review.
Instructor

Offered by
Explore more from Machine Learning
- Status: Free Trial
University of Glasgow
- Status: Free Trial
University of Glasgow
- Status: Free Trial
- Status: Free Trial
LearnQuest
Why people choose Coursera for their career





Open new doors with Coursera Plus
Unlimited access to 10,000+ world-class courses, hands-on projects, and job-ready certificate programs - all included in your subscription
Advance your career with an online degree
Earn a degree from world-class universities - 100% online
Join over 3,400 global companies that choose Coursera for Business
Upskill your employees to excel in the digital economy
Frequently asked questions
Access to lectures and assignments depends on your type of enrollment. If you take a course in audit mode, you will be able to see most course materials for free. To access graded assignments and to earn a Certificate, you will need to purchase the Certificate experience, during or after your audit. If you don't see the audit option:
The course may not offer an audit option. You can try a Free Trial instead, or apply for Financial Aid.
The course may offer 'Full Course, No Certificate' instead. This option lets you see all course materials, submit required assessments, and get a final grade. This also means that you will not be able to purchase a Certificate experience.
When you enroll in the course, you get access to all of the courses in the Specialization, and you earn a certificate when you complete the work. Your electronic Certificate will be added to your Accomplishments page - from there, you can print your Certificate or add it to your LinkedIn profile. If you only want to read and view the course content, you can audit the course for free.
If you subscribed, you get a 7-day free trial during which you can cancel at no penalty. After that, we don’t give refunds, but you can cancel your subscription at any time. See our full refund policy.
More questions
Financial aid available,