In this course, we’re going to go over analytical solutions to common healthcare problems. I will review these business problems and you’ll build out various data structures to organize your data. We’ll then explore ways to group data and categorize medical codes into analytical categories. You will then be able to extract, transform, and load data into data structures required for solving medical problems and be able to also harmonize data from multiple sources. Finally, you will create a data dictionary to communicate the source and value of data. Creating these artifacts of data processes is a key skill when working with healthcare data.

Heat up your career with 40% off top courses from Google, Adobe, and more. Save today.
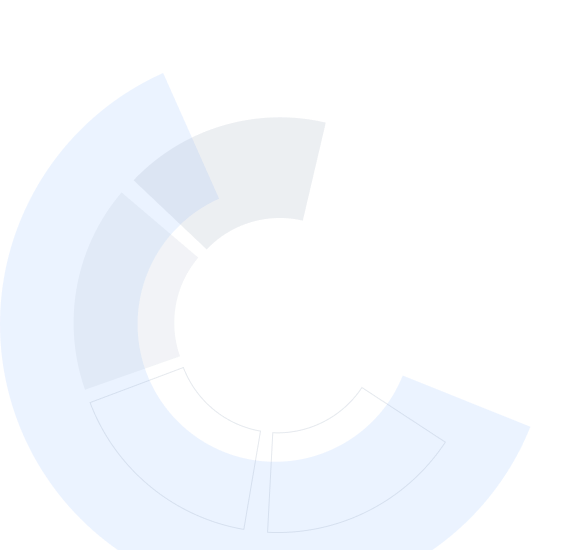
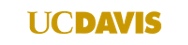
Analytical Solutions to Common Healthcare Problems
This course is part of Health Information Literacy for Data Analytics Specialization

Instructor: Brian Paciotti
6,054 already enrolled
Included with
(33 reviews)
Skills you'll gain
Details to know

Add to your LinkedIn profile
4 assignments
See how employees at top companies are mastering in-demand skills
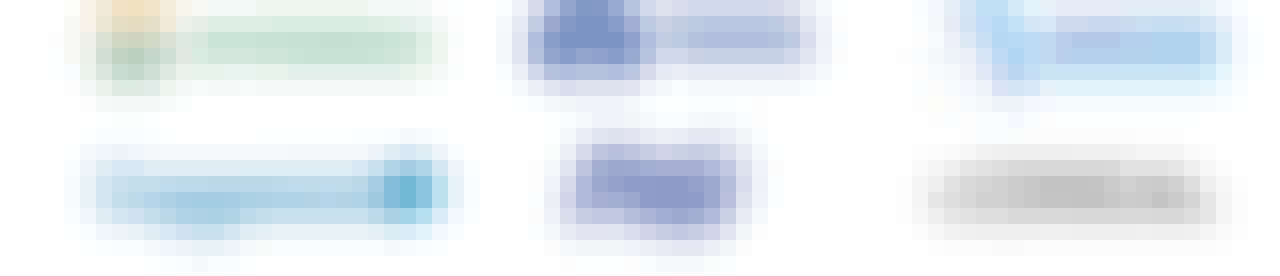
Build your subject-matter expertise
- Learn new concepts from industry experts
- Gain a foundational understanding of a subject or tool
- Develop job-relevant skills with hands-on projects
- Earn a shareable career certificate
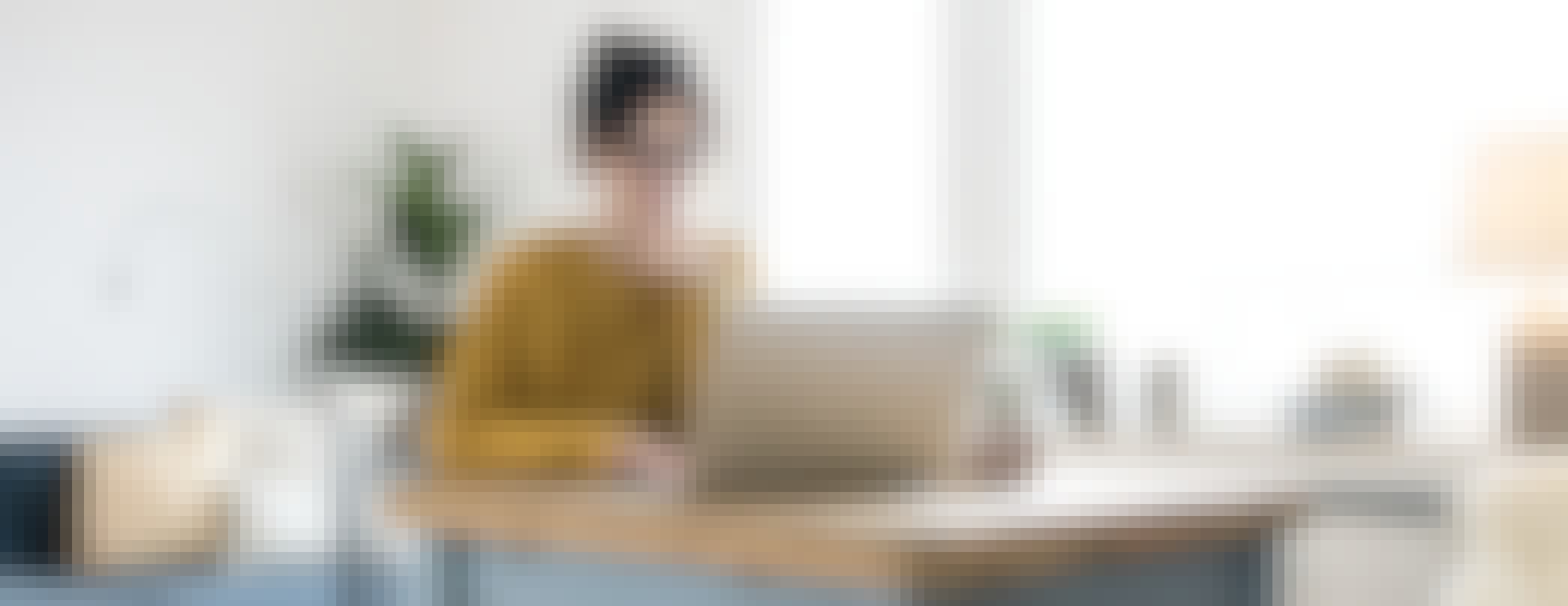
There are 4 modules in this course
In this module, you will explain why comparing healthcare providers with respect to quality can be beneficial, and what types of metrics and reporting mechanisms can drive quality improvement. You'll recognize the importance of making quality comparisons fairer with risk adjustment and be able to defend this methodology to healthcare providers by stating the importance of clinical and non-clinical adjustment variables, and the importance of high-quality data. You will distinguish the important conceptual steps of performing risk-adjustment; and be able to express the serious nature of medical errors within the US healthcare system, and communicate to stakeholders that reliable performance measures and associated interventions are available to help solve this tremendous problem. You will distinguish the traits that help categorize people into the small group of super-utilizers and summarize how this population can be identified and evaluated. You'll inform healthcare managers how healthcare fraud differs from other types of fraud by illustrating various schemes that fraudsters use to expropriate resources. You will discuss analytical methods that can be applied to healthcare data systems to identify potential fraud schemes.
What's included
8 videos1 reading1 assignment3 discussion prompts
In this module, you will define clinical identification algorithms, identify how data are transformed by algorithm rules, and articulate why some data types are more or less reliable than others when constructing the algorithms. You will also review some quality measures that have NQF endorsement and that are commonly used among health care organizations. You will discuss how groupers can help you analyze a large sample of claims or clinical data. You'll access open source groupers online, and prepare an analytical plan to map codes to more general and usable diagnosis and procedure categories. You will also prepare an analytical plan to map codes to more general and usable analytical categories as well as prepare a value statement for various commercial groupers to inform analytic teams what benefits they can gain from these commercial tools in comparison to the licensing and implementation costs.
What's included
7 videos1 assignment1 discussion prompt
In this module, you will describe logical processes used by database and statistical programmers to extract, transform, and load (ETL) data into data structures required for solving medical problems. You will also harmonize data from multiple sources and prepare integrated data files for analysis.
What's included
6 videos1 assignment1 discussion prompt
In this module, you will describe to an analytical team how risk stratification can categorize patients who might have specific needs or problems. You'll list and explain the meaning of the steps when performing risk stratification. You will apply some analytical concepts such as groupers to large samples of Medicare data, also use the data dictionaries and codebooks to demonstrate why understanding the source and purpose of data is so critical. You will articulate what is meant by the general phase -- “Context matters when analyzing and interpreting healthcare data.” You will also communicate specific questions and ideas that will help you and others on your analytical team understand the meaning of your data.
What's included
7 videos1 reading1 assignment1 peer review2 discussion prompts
Earn a career certificate
Add this credential to your LinkedIn profile, resume, or CV. Share it on social media and in your performance review.
Instructor

Offered by
Explore more from Health Informatics
Northeastern University
- Status: Free Trial
University of California, Davis
- Status: Free Trial
University of Colorado Boulder
- Status: Free Trial
University of California, Davis
Why people choose Coursera for their career




Learner reviews
33 reviews
- 5 stars
60.60%
- 4 stars
27.27%
- 3 stars
6.06%
- 2 stars
6.06%
- 1 star
0%
Showing 3 of 33
Reviewed on Jan 5, 2020
Very good, although I would suggest the Health Informatics as a starting course
Reviewed on Jun 25, 2020
Very informative. Would have preferred more practical examples on data analysis
Reviewed on Jan 17, 2020
Excellent material and a great introduction to data analytics!

Open new doors with Coursera Plus
Unlimited access to 10,000+ world-class courses, hands-on projects, and job-ready certificate programs - all included in your subscription
Advance your career with an online degree
Earn a degree from world-class universities - 100% online
Join over 3,400 global companies that choose Coursera for Business
Upskill your employees to excel in the digital economy
Frequently asked questions
Access to lectures and assignments depends on your type of enrollment. If you take a course in audit mode, you will be able to see most course materials for free. To access graded assignments and to earn a Certificate, you will need to purchase the Certificate experience, during or after your audit. If you don't see the audit option:
The course may not offer an audit option. You can try a Free Trial instead, or apply for Financial Aid.
The course may offer 'Full Course, No Certificate' instead. This option lets you see all course materials, submit required assessments, and get a final grade. This also means that you will not be able to purchase a Certificate experience.
When you enroll in the course, you get access to all of the courses in the Specialization, and you earn a certificate when you complete the work. Your electronic Certificate will be added to your Accomplishments page - from there, you can print your Certificate or add it to your LinkedIn profile. If you only want to read and view the course content, you can audit the course for free.
If you subscribed, you get a 7-day free trial during which you can cancel at no penalty. After that, we don’t give refunds, but you can cancel your subscription at any time. See our full refund policy.
More questions
Financial aid available,